You are here
Art and archaeology are the new frontiers for AI
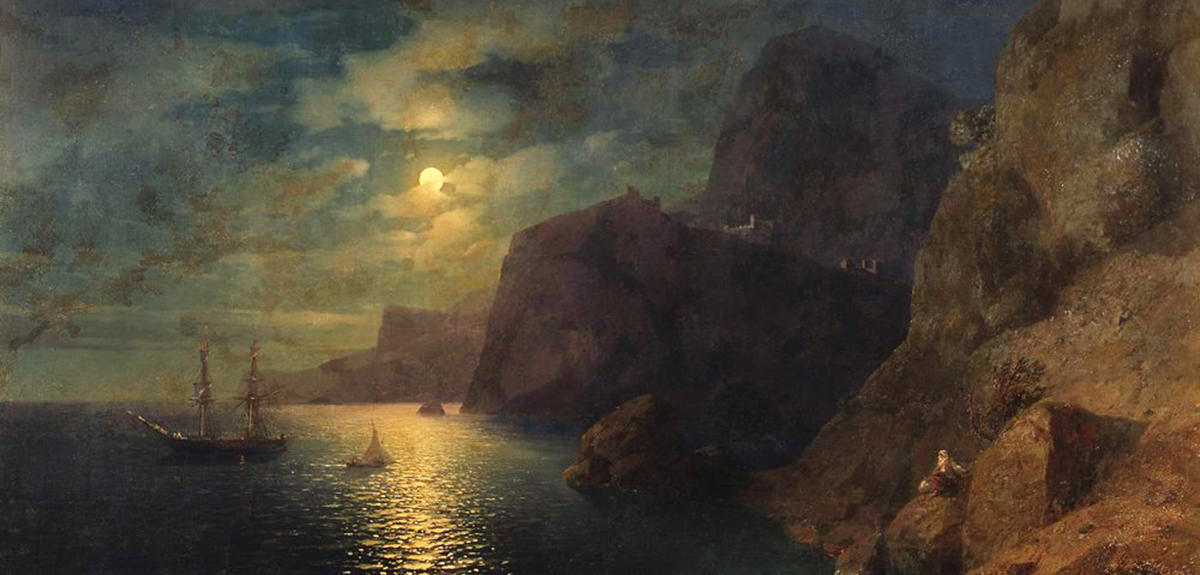
In the past few years, artificial intelligence (AI) has become an essential tool for sorting and managing the profusion of images that circulate online. We are now accustomed to the idea of algorithms that can recognise the presence of faces or distinguish between a dog and a cat. The next step will be to take AI beyond such simple, prosaic descriptions. Maks Ovsjanikov, a professor at the LIX,1 who specialises in the study of 3D and image data, is now developing algorithms that offer archaeologists and art historians automated, reproducible solutions for analysing artefacts and artwork.
Classifying prehistoric mandibles
“In concrete terms, my job is to find similarities and differences between three-dimensional objects, and to fill in any missing pieces,” explains Ovsjanikov, winner of the 2018 CNRS Bronze Medal. The young researcher was approached by scientists working at a major prehistoric site: the Arago Cave, in southwestern France, where Tautavel Man was discovered. The team, which includes the prehistorians Henry and Marie-Antoinette de Lumley, was having problems with the identification of ten human mandibles. Unearthed at a site of human occupation dating back some 600,000 years, the bones are indeed too old to contain DNA and too decomposed to be attributed with certainty to one of the many species of the Homo genus that lived over successive millennia in and around the cave.
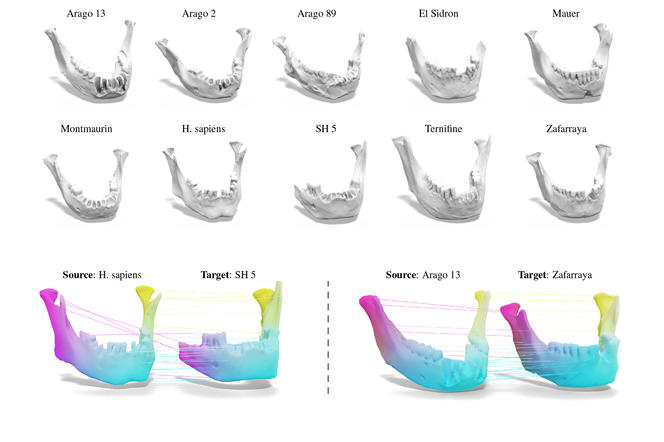
The researchers already had extensive 3D data on the mandibles, which Ovsjanikov and his team used in an attempt to identify, based on algorithms, similarities and differences in shape that might be difficult to detect and could have eluded the prehistorians’ expert eyes. This approach makes the analysis more efficient, eliminating the bias that can arise in subjective annotation, even when conducted by a specialist. “Rather than the time-consuming process of marking points of interest in the 3D data by hand, I offer a reliable automatic method based on unsupervised learning,” Ovsjanikov explains. “This approach also ‘wipes out’ the biaising effects that appear on the geometry of the bones when these are run through the scanner. The Tautavel project is nonetheless quite difficult, because there are not enough mandibles to establish a statistical base. The work isn’t finished yet, but we’ve already taken a big step in the right direction.”
An emotional Turing test
Ovsjanikov also works with two-dimensional images. In cooperation with colleagues from Stanford University (California, US) and King Abdullah University of Science and Technology (KAUST) in Saudi Arabia, he has launched the ArtEmis project, with the goal of teaching algorithms to interpret emotional responses to artwork. “I’m trying to identify and quantify what makes an object unique – it’s nearly a philosophical question,” Ovsjanikov says with a smile. “Things are relatively simple when it’s just a matter of describing the content of a photograph, but how can a system understand a work of art? And what if it is abstract? We need an in-depth analysis of the subjective relation between the image and the viewer.”
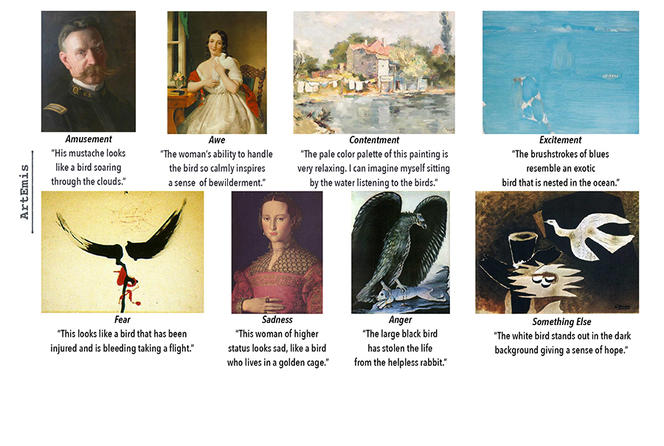
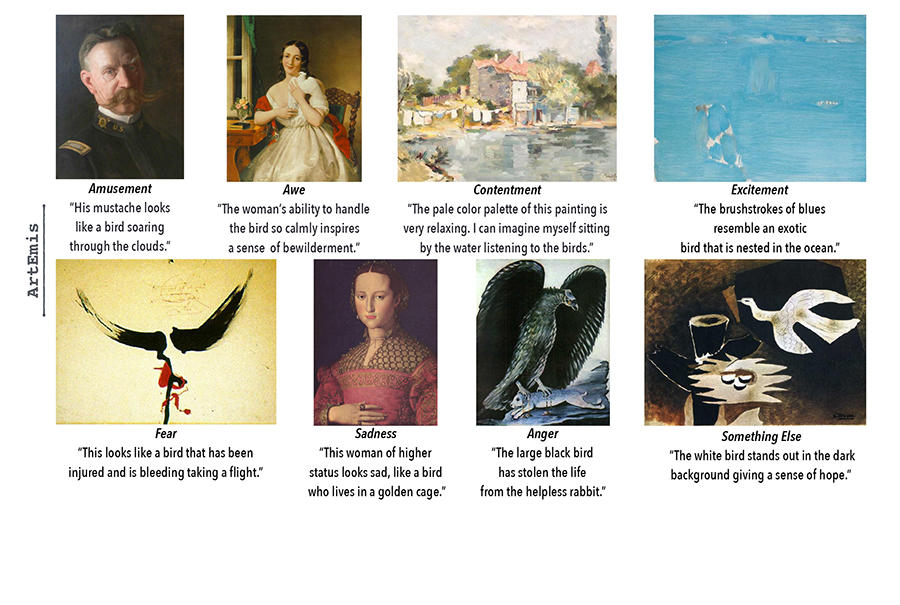
In order to have a large enough data set, the researchers worked with a huge panel of volunteers, compensated through a microwork service platform. The participants were asked to describe their predominant emotional sensations when viewing various artworks and to explain the reasons for their reactions.
Many of the responses arose from the images’ subjective impact – “It makes me sad/happy” – or from a continuum of thought: “There is a bird, birds make me think of freedom, so the painting brings to mind freedom.” Some 455,000 entries were compiled concerning 90,000 works of art. This data was then used to train the algorithms, enabling them to comment on the artwork as a human viewer would. The system was then subjected to new images that had not previously been presented to the participants in the panel.
These answers were then integrated into a survey – or what the researchers call an “emotional Turing test” – in which people were asked to decide whether an analysis was of algorithmic or human origin. Half of the time, the respondents were not able to distinguish the AI system’s statements from those of human viewers.
Towards robot art experts?
No direct applications for these results have been found in the short term, but the researchers envision future methods for modifying images in order to give them the desired emotional impact. Contemporary artists who use algorithms to generate works more or less automatically could also benefit from this type of research. “The world is not simply a list of the objects that we see,” Ovsjanikov emphasises. “We must also consider the effect that they have on us. If we want to create robots and algorithms capable of communicating like human beings, we need to integrate different forms of subjectivity. And very little work has actually been done on these complex questions so far.”
Pending the development of practical applications, Ovsjanikov and his team are also investigating the biomedical sector. The intention is not to replace the expertise and decision-making capacities of a physician, but shape data analysis can be an aid in diagnosis, differentiating types of organic tissues, or analysing a tumour. “It’s very gratifying to find ways of working in symbiosis with other researchers, developing automatic methods that are useful to them,” Ovsjanikov concludes. “The quality and quantity of 3D data resulting from research are constantly increasing. Now we have to give meaning to this data.”
- 1. Laboratoire d’Informatique de l’École Polytechnique (CNRS / École Polytechnique).
Keywords
Share this article
Author
A graduate from the School of Journalism in Lille, Martin Koppe has worked for a number of publications including Dossiers d’archéologie, Science et Vie Junior and La Recherche, as well the website Maxisciences.com. He also holds degrees in art history, archaeometry, and epistemology.
Comments
Log in, join the CNRS News community