You are here
AI detects the early signs of multiple sclerosis
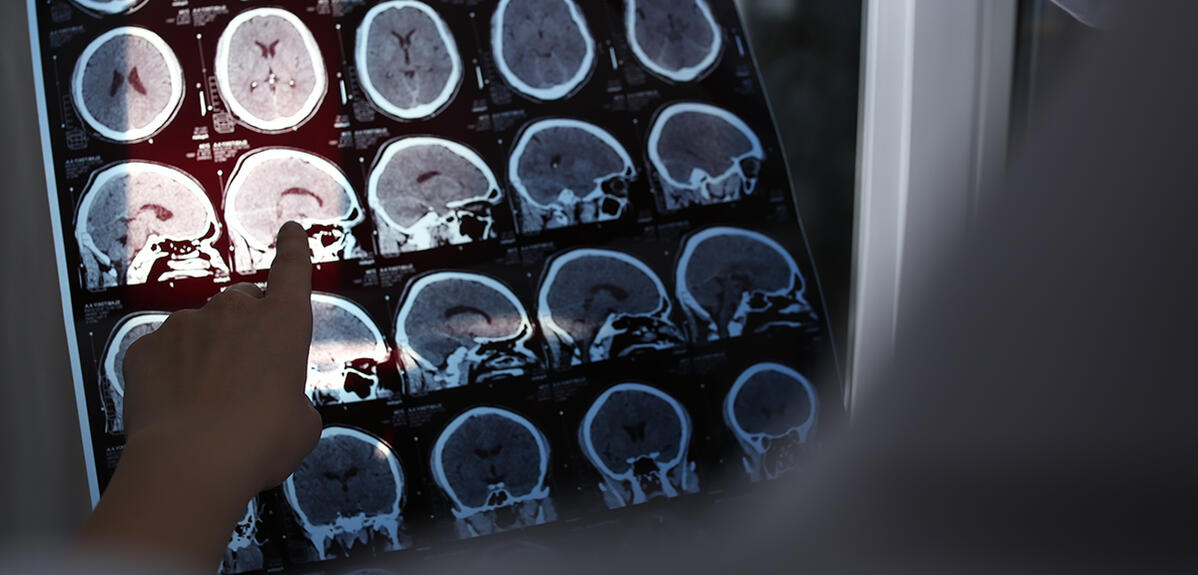
It mainly appears in young adults between the ages of 25 and 35 years, and may concern more than 115,000 people in France, with 5,000 new cases being diagnosed each year1. Multiple sclerosis (MS) is an inflammatory, autoimmune and neurodegenerative disease that affects the brain and spinal cord. The inflammation destroys myelin, which can then no longer fulfill its role as a sheath to protect neuronal axons. This loss particularly impacts the transmission of information passing between nerve cells. This chronic condition impairs motor, visual, sensory and cognitive functions, depending on the size and site of the lesions it has inflicted. Treatments are available to restrict its progression, but it cannot yet be cured.
Mapping changes to the volume of brain structures
The study and monitoring of multiple sclerosis are complicated by the simple fact that patients only undergo MRI (magnetic resonance imaging) scans once they have experienced the initial symptoms. This is a recurrent problem with neurodegenerative diseases, where several brain structures are already affected without it being known when or how it started.
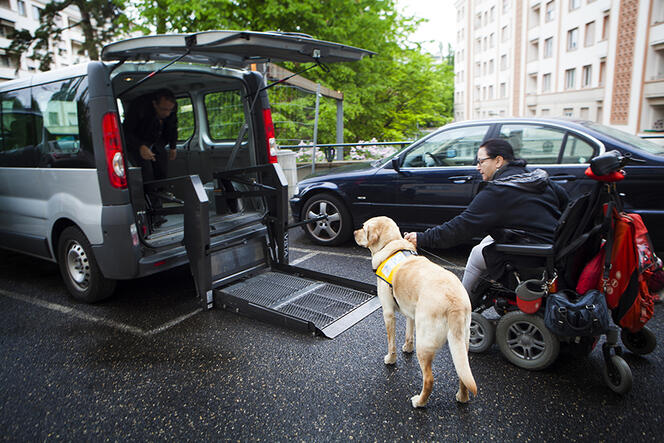
This puzzle does not deter Pierrick Coupé, CNRS research professor in the LaBRI2, who works on the analysis and processing of biomedical images in the context of neurodegenerative diseases such as MS or Alzheimer’s. Since 2019, this scientist has been focusing on the development of so-called “lifespan” models, an approach that makes it possible to situate any phenomena chronologically. “No-one undergoes MRI scans on a regular basis from birth to death, so the models have been built using tens of thousands of images of the brains of different individuals of all ages,” he explains. “This makes it possible to estimate mean trajectories for the volumes of brain structures throughout life, in the same way as the curves used to monitor height and weight in children.”
This work, described in the journal Human Brain Mapping, was initially intended to investigate normal ageing of the brain. But Coupé and his colleagues diverted the method to studying conditions such as Alzheimer’s. They were thus able to show that curves for the volume of the hippocampus, a structure essential to memory, started to diverge from those of normal ageing from the age of forty3.
Massive processing of medical images
For multiple sclerosis, the scientists analysed the MRI brain scans of almost 41,000 subjects, including more than 2,500 patients from the French Ofsep observatory on multiple sclerosis database. “MS tends to be described as an inflammatory condition, but we looked at it from the angle of neurodegeneration,” says Coupé. “We wanted in particular to check whether the disease affected the brain structures early and specifically.”
The aim was therefore to identify all regions of the brain and monitor the evolution of their volume during the lifespan of individuals, whether they suffered from MS or not. So the purpose was no longer to develop a tool, but rather to create knowledge. “A detailed segmentation of the brain using MRI images requires two weeks of work by a specially trained person,” the researcher regrets. “With 41,000 brains to analyse, this would have been completely impossible without AI.”
The scientists therefore developed collective AI where numerous networks of neurons work in sync in order to converge towards a precise and robust result. As it happens, no fewer than 250 neural networks analysed the image at the same time. This approach was borrowed from the method used by humans to process complex subjects. “We drew our inspiration from a two-chamber parliamentary system,” explains Coupé. “The two neuron networks are organised like these chambers: one conducts a low-resolution analysis while the other refines its results. They communicate throughout the process until they reach a consensus resulting in the discrimination of different brain structures. The major problem with managing a large amount of data is the control of its quality, and some of the neuron networks are solely dedicated to verifying the integrity of these analyses”.
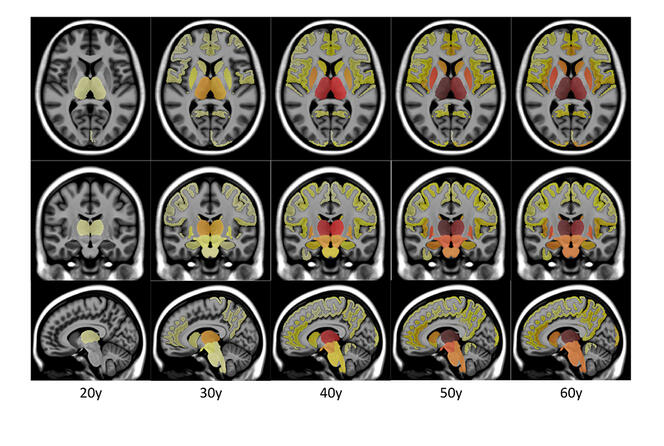
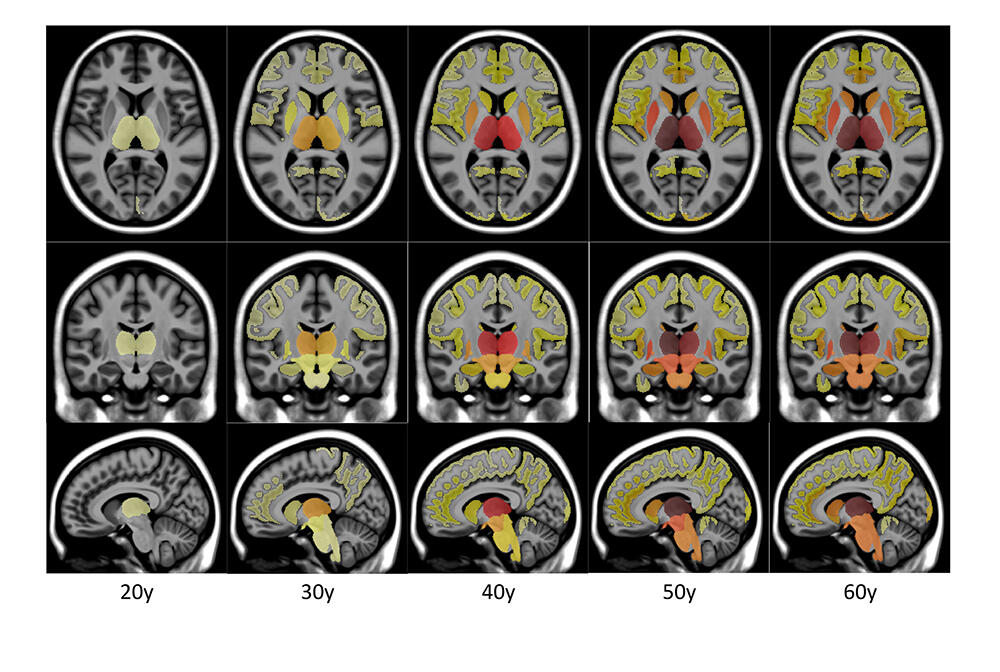
AI was able to segment the brain and then process the data from 41,000 subjects thus obtained. These segmentations made it possible to infer the trajectory over life of the average evolution of the volume of each brain structure, for which both a normal and a pathological trajectory were constructed in order to estimate the point at which the two models diverged.
This work showed that the thalamus, involved in processing sensitivity and sensory data, was the first structure to be impacted by multiple sclerosis. The putamen, which plays a role in regulating movement and learning, generally starts to deteriorate some four years later. As for the brain stem, which notably regulates heart rate and respiration, it was affected around nine years after the thalamus. In other words, the results obtained by Coupé and his colleagues have shown that several brain structures clearly suffered damage well before the appearance of the first symptoms of multiple sclerosis, which on average were detected more than a decade after the initial signs of neurodegeneration.
Conclusions that can be explained
These results underline the important role played by artificial intelligence in the study and diagnosis of various pathologies, thanks to the mass processing of medical imaging findings. The fact that this work was based on IA using brain segmentation understandable by humans is important, because it means that the conclusions can be explained. Indeed, numerous AI systems achieve apparently correct results but there is no access to their pathways, which limits the chances of detecting a problem if they are mistaken. It is also clearly unadvisable to make a diagnosis based on algorithms that cannot clarify or justify their choices.
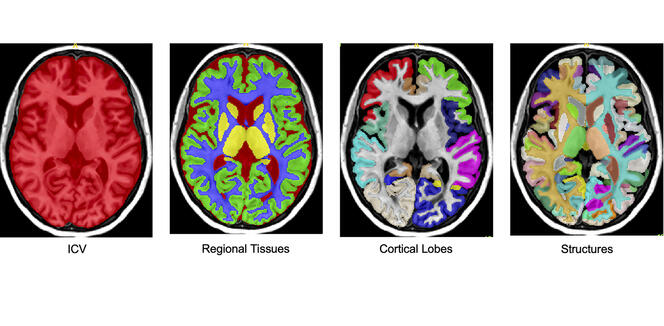
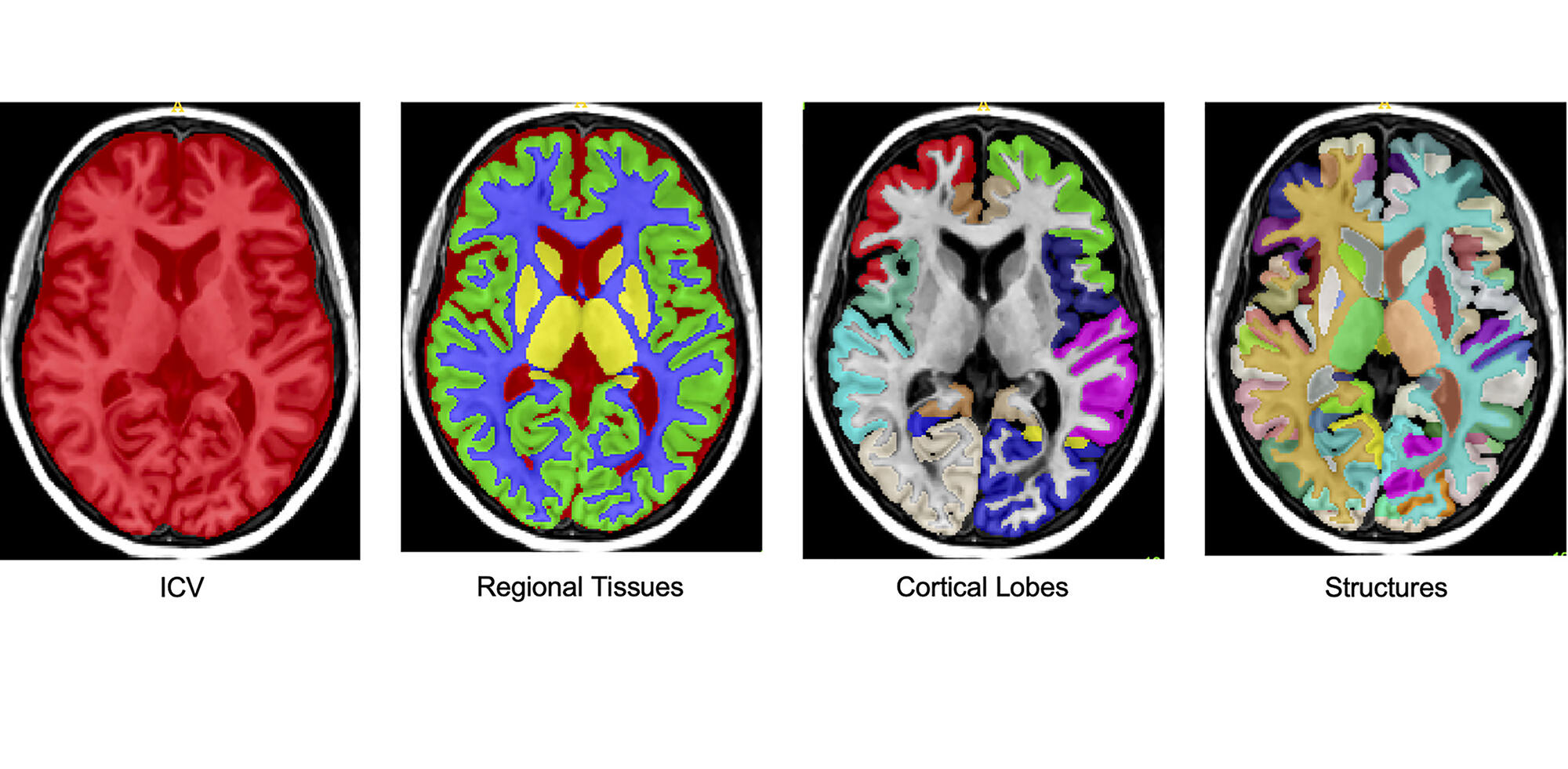
“We have recently developed a diagnostic support tool for Alzheimer’s disease4, because we found that two structures diverged strongly and at an early stage,” Coupé adds. “We are currently working on a similar device for multiple sclerosis. Furthermore, our models can reduce the costs of clinical studies by helping to better select the patients included. In a more distant future, we hope to design AI tools that can provide diagnostic support to differentiate between several types of dementia, such as Alzheimer’s disease or frontotemporal dementia”.♦
Go to our website:
https://news.cnrs.fr/articles/ia-leads-the-charge-against-multiple-sclerosis
- 1. https://www.arsep.org/fr/159-la-sep.html (in French).
- 2. Laboratoire Bordelais de Recherche en Informatique (CNRS / Bordeaux INP / Université de Bordeaux).
- 3. https://onlinelibrary.wiley.com/doi/full/10.1002/hbm.26464.
- 4. onlinelibrary.wiley.com/doi/10.1002/hbm.25850.
Keywords
Share this article
Author
A graduate from the School of Journalism in Lille, Martin Koppe has worked for a number of publications including Dossiers d’archéologie, Science et Vie Junior and La Recherche, as well the website Maxisciences.com. He also holds degrees in art history, archaeometry, and epistemology.
Comments
Log in, join the CNRS News community