You are here
Could Artificial Intelligence Overhaul Healthcare?
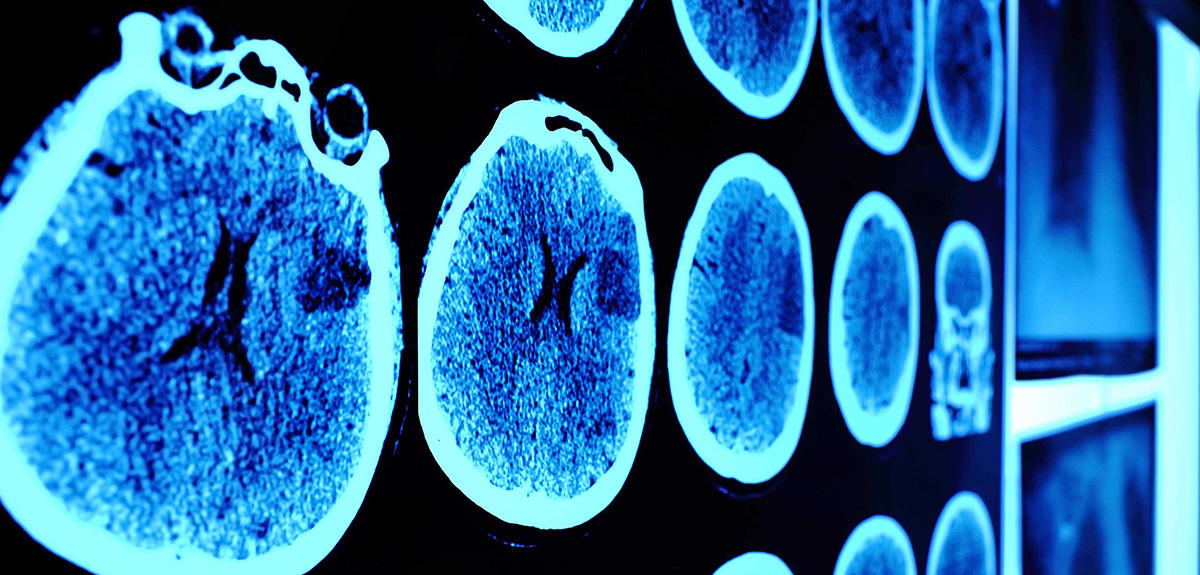
“Almost all fields of artificial intelligence have applications in healthcare.”1 Medicine appears to have entered the era of data, and artificial intelligence (AI) will prove a valuable tool in the future, notably as an aid to diagnosis.
Watson, the program developed by IBM, is the most emblematic example. Based on deep learning, the best known branch of artificial intelligence, it operates by layers, like a network of interconnected neurons spread between different strata for each calculation. The answer is only “produced” after a learning process which from the start associates symptoms and pathology. It is wholly calculated from statistical analysis and consists of probabilities that the response will be correct. Watson learns independently from a gigantic corpus of knowledge, and thus creates its own connections autonomously as it progresses. However, this type of artificial intelligence has a major drawback: it operates like a "black box" and no-one can explain the results, which in turn will also enrich the initial corpus.
An autonomous learning loop
“Relying on the medical knowledge it possesses, the machine acquires further expertise in the same way as a human being; the more it knows, the more it learns,” explains Amedeo Napoli, senior researcher at the LORIA.2 His team is "mining medical data which is fed to an intelligent system and thus creates an autonomous learning loop."


The aim is to screen a whole range of metabolic information so as to enable the emergence of markers that can predict and discriminate type 2 diabetes, or new antibiotic compounds able to combat bacterial resistance. Determining correlations between molecular elements and certain functional properties of compounds may lead to the discovery of new molecular structures. Based on these⎯sometimes unexpected⎯correlations, operational and effective drugs may see the light of day.
“We have also developed a decision-support system for oncologists, which operates by analogy with a standard case of a cancer patient,” adds the scientist. Care paths will also be better monitored, because "although an oncologist can learn a dozen of them, a machine will contain years of management data.” This decision-support system was designed by cancer specialists and clinicians, as is almost always the case for systems used in a particular field.
Refining a diagnosis and predicting its course
In medical imaging, the AI revolution may prove colossal. In this case, the machine needs to be trained in detecting particular characteristics and regular properties, such as the shape and volume of different areas of the brain. The aim is to observe these regular features and then follow their evolution over time and in different patients. To achieve this, the system sorts and classifies a large number of brain images. Each of them is associated⎯or not⎯with a pathological condition, which makes it possible to automatically place patients in diagnostic categories. Depending on how they develop, the longitudinal database will be enriched: which areas have changed? Which patients have developed Alzheimer’s? How long did it take? All this data will subsequently be crossed in the hope of “predicting the course of neurodegenerative diseases such as Alzheimer’s at very early stages, ten or twenty years before onset of the first symptoms,” comments Olivier Colliot, head of the Aramis team at the ICM.3
“As a general rule, radiologists are quite good at making a diagnosis at time t, but less so when it comes to the prognosis,” adds the scientist. Yet are these specialists destined to disappear? “I don’t think so, but their work will certainly change. It will no longer be a simple visual analysis, as it will involve predictions and decisions based on combining the images and data supplied by an artificial intelligence system,” Colliot believes.
This will be all the more necessary as these systems are restricted to ultra-specialized tasks. For example, if the machine has been tuned to detect the brain lesions of Alzheimer’s disease, it will not be able to find a tumor that would otherwise be obvious to a radiologist. “Human beings still have the advantage of considerable contextual knowledge that a machine cannot grasp,” insists the scientist.
In some cases, however, the performance of a machine can exceed that of a physician. In January 2017, an artificial intelligence system supplied by Google, called Show and Tell and based on image classification, succeeded in detecting 90% of benign skin blemishes, versus 76% among the dermatologists questioned (out of 130,000 images analyzed). And "in Stanford, a team showed that artificial intelligence performed better for detecting diabetic retinopathy than ophthalmologists,” reveals José-Alain Sahel, professor of ophthalmology and director of the Paris-based Institut de la Vision.4 “Artificial intelligence in medicine is approaching the performance of the game of Go," he suggests.
Simulating is not gaming
Young physicians are not being left out. A serious game, or simulator which "uses gaming methods to teach how to conduct a consultation with a patient" has thus been developed by Pierre Zweigenbaum and his team at the LIMSI5 in Orsay. Placed before an avatar that explains its symptoms, medical history and lifestyle during a mock examination, students must interact with AI by typing in their responses.
To develop this device, the scientists used supervised learning, one of the multiple facets of artificial intelligence. “The first stage is completed by a human being, as the machine needs to be taught the different associations between input and output data,” explains Zweigenbaum.
As a brief example, this might require associating pain in the lower left quadrant with a possible attack of appendicitis, and so on for all types of symptoms and pathologies. The second stage consists in presenting new cases to the machine "without giving it solutions!” The data input and output pathways are therefore only provided once.
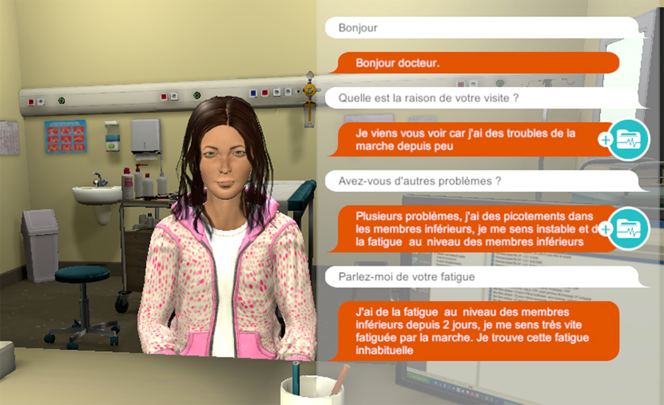
At a more mundane level, artificial intelligence should considerably facilitate the administrative management of hospitals. “Once there is language or text, artificial intelligence can be produced,” explains Zweigenbaum. “Language is the vehicle of knowledge." Beyond a simple technology, this intelligence questions our relationship with language and knowledge, and can sometimes shed light on patient-physician relationships. When developing his simulator, Zweigenbaum was surprised by "the breadth of the vocabulary used in medicine, the accuracy with which one can describe a pain or a respiratory problem.” How can this learning, sometimes based on the subjective experiences of patients, be rationalized by AI? Scientific publications, physicians and patients have different levels of comprehension that must be integrated by the machine. Zweigenbaum was faced with this complexity when the French Medicines Agency (ANSM) asked him to analyze medical forums on the Internet in order to conduct pharmacovigilance. “The language used by patients on the web is very different from medical vocabulary. Some medicines even have nicknames,” he adds.
Towards a paradigm shift in medicine?
These intelligent systems are thus opening up new research opportunities. “We shall be seeing unexpected correlations; for example, when a compound developed in another field may have beneficial effects on age-related macular degeneration,” points out Sahel.
These systems may become more rapid in establishing correlations between genes, diagnoses, symptoms and the environment by processing a huge amount of disorganized scientific data, and driving medical research towards a paradigm shift. While the conventional research methodology starts from a hypothesis to be explored, it may now be sufficient to analyze the data and see what emerges. The “omic” sciences, such as genomics or proteomics, are predicting increasingly personalized medicine based on artificial intelligence. Yet it remains to be determined whether these technological advances will be pertinent and accessible to all.
Accumulating information is useful, but it needs to be reliable in order to develop efficient intelligent systems, "particularly when this involves separate groups of data collected in many countries,” notes Sahel. “We have powerful machines, but the data is becoming increasingly complex and in certain cases cannot be exploited,” adds Napoli. Some medical fields still lack samples to develop these systems. “In cerebral imaging, we often have only around a thousand patients with a particular condition on which to base our work,” emphasizes Colliot. Zweigenbaum, on the other hand, advocates that health data should be more available to research scientists “although this would obviously have to be controlled.”
Humans or machines: whose fault is it?
Responsibility is of course a crucial issue. Man or machine, who makes the medical error? “For driverless cars, it is possible to imagine that there will no longer be any human intervention, but in medicine, the final decision will always be down to the clinician,” Colliot believes. Radiologists, ophthalmologists, or oncologists, all are responsible, because they can call into question the data delivered by the machine. And it is always a physician who makes the final choice regarding patient management and the prescription of medication. In that respect, artificial intelligence provides a simple decision-support tool in the same way as a microscope or stethoscope. If the resource fails, the clinician will certainly remain wholly responsible.
Furthermore, patients’ acceptance has not yet been obtained and these systems raise personal data protection issues. Thus, in July 2017, Google, owner of the artificial intelligence tool DeepMind Health, was censured for having divulged information on the health of more than 1.5 million Londoners, after a contract had been signed between the firm and the UK health authorities. The aim of the system was to develop an application to monitor patients with renal failure, but the latter were little aware of the use of their medical records in the long term, which amounted to a lack of transparency according to the UK Information Commissioner’s Office.
In Napoli’s opinion, AI should become a "real intelligent assistant" for physicians, rather than replace them. “This decision support will certainly transform the work of clinicians,” adds Colliot. In addition, medicine is not just about diagnosis and technology. As early as 1973, Georges Canguilhem, an expert in the philosophy of medicine, was questioning the consequences for patients: "Will diagnosis and treatment by a computer meet all of a patient’s needs? Aren’t the presence of a living being, a message of life also necessary?” Medicine is based on human relationships, and "these are irreplaceable," concludes Sahel. "Artificial intelligence will not change that, but it will reduce the proportion of human failure.”
- 1. Conclusion to the report on the AI Strategy for France: http://www.enseignementsup-recherche.gouv.fr/cid114739/rapport-strategie...
- 2. Laboratoire Lorrain de Recherche en Informatique et ses Applications (CNRS / Inria / Université de Lorraine).
- 3. Institut du Cerveau et de la Moelle Épinière (CNRS / Inserm / Sorbonne Université / APHP).
- 4. CNRS / UPMC / Inserm.
- 5. Laboratoire d’Informatique pour la Mécanique et les Sciences de l’Ingénieur (CNRS / Université Paris-Saclay).
Explore more
Author
Léa Galanopoulo has a biology degree and is currently studying scientific journalism at Paris-Diderot university.