You are here
Athénan, a multi-champion AI
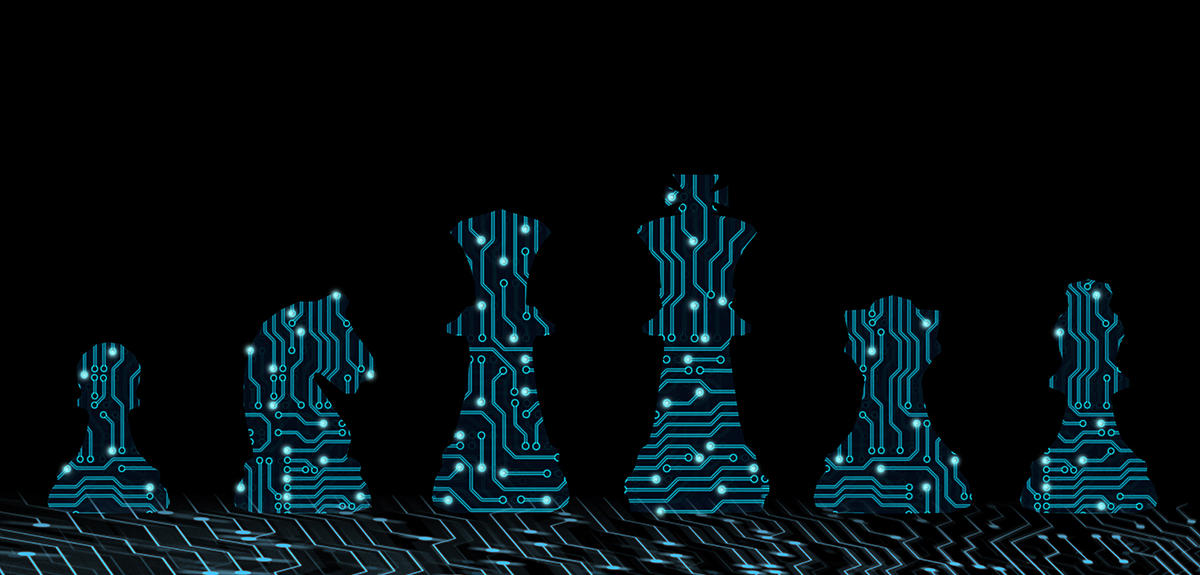
Athénan is a new artificial intelligence system (AI) designed for board games that was created by Quentin Cohen-Solal,1 and subsequently evaluated and improved by Tristan Cazenave at the LAMSADE2 in Paris. At the 24th Computer Olympiad, Athénan earned its name – which literally means "disciple of Athena" (the Greek goddess of strategy and victory) – at the games of Canadian and Brazilian draughts, Othello, Hex, and Surakarta. It beat all competitors at 11 of the 23 board games represented, winning just as many gold medals! "Usually, the best teams earn 2 or 3 gold medals. This goes to show the advances that this work enabled," says Nicolas Jouandeau, director of the LIASD advanced computer science laboratory at Saint-Denis Université (Paris).
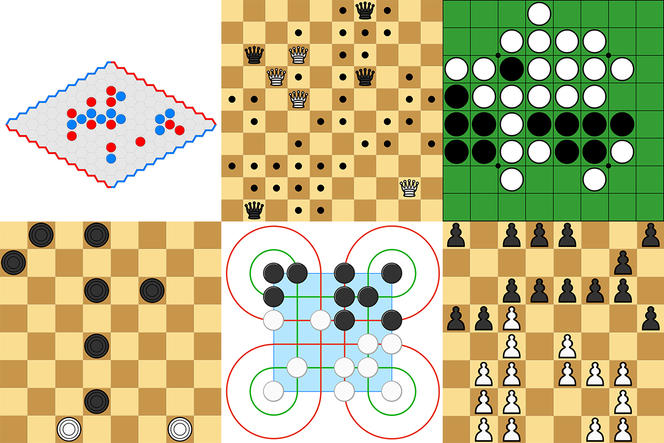
AI systems designed for board games consist of algorithms that formalise a way of thinking about a given game, and even how to learn and practice on their own, as in the case of Athénan. The development of such software programs has always attracted AI experts, and for good reason; board games, or "strategy games", involve a broad range of human intellectual capacities, and offer a privileged field of experimentation for testing machine intelligence and comparing it to human intelligence, with one of AI’s goals being the development of devices that outperform (and thereby replace) the human brain for certain specific tasks.
A quest that dates back to the beginnings of computer science
"The idea of developing AI designed for board games emerged in the early days of computer science," points out Cazenave. In the late 1940s, the founding fathers of the discipline, Alan Turing (1912-1954) and Claude Shannon (1916-2001), proposed basic programs that could play chess. "Yet it was only in 1997, nearly 40 years later, that a machine – the Deep Blue supercomputer from the American company IBM – beat a world chess champion, Garry Kasparov of Russia, for the first time under the normal conditions of a tournament."

In May 2017, nearly two decades after this first masterstroke, another AI program named AlphaGo3, which was developed by DeepMind (a British subsidiary of Google) and designed for the game of Go (a Chinese strategy game), beat the world number one, Ke Jie of China. "AlphaGo uses radically different techniques from those of Deep Blue, namely 'deep' neural networks, one of the most recent technological developments in AI. These networks are made up of a system that was, at the outset, schematically inspired from the functioning of human neurons. This technology allows AlphaGo to learn how to 'imitate' human players using a database that contains moves from tens of thousands of matches played by expert players," explains Cazenave.
The era of "universal" AI
In late 2017, the company DeepMind presented AlphaZero,4 another historic advance in AI. The distinctive feature of this "universal" version of AlphaGo can play multiple games – not just Go, but chess and Shogi (Japanese chess) as well. "Unlike its predecessor, AlphaZero was developed to learn how to play a game by playing against itself, rather than relying on a database," the researcher points out. "It uses a reinforcement learning method to analyse its successes and errors after each game, in order to improve."
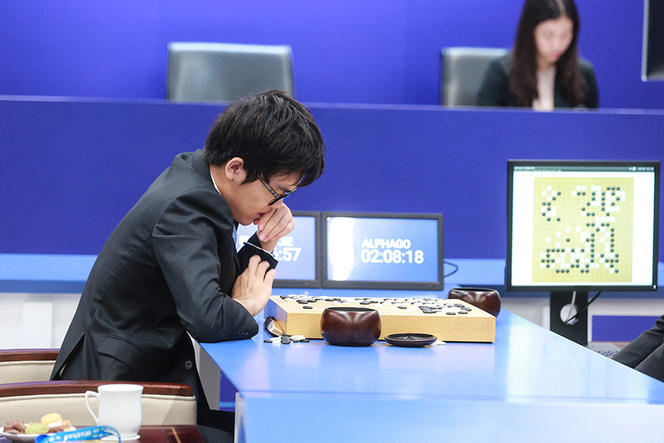
Athénan is the second universal system based on this technique. "It differs from AlphaZero in a number of respects that make it original and highly effective," emphasises Cohen-Solal. While Athénan also uses a neural network to guide its search for a strategy during a match, as AlphaZero does, it employs a different algorithm to anticipate future moves. AlphaZero resorts to what is known as the Monte Carlo tree search method, which generates statistics based on random games (hence its name evoking the famous casino): for each move analysed, the neural network calculates the value of a "game state" (assessing how near it is to victory), as well as the probability that a move is the best one given that state. "That is not how the human mind works," the researcher observes.
A more human way to play
Hence his idea to develop "a method allowing AI to play in a more human manner, known as 'unbounded best-first Minimax'. Thanks to this algorithm – described for the first time in 19965 but forgotten until I rediscovered it – Athénan does not calculate or use probabilities, with no negative impact on its performance".
Another major advantage of this method is that it allows for more effective learning: "While AlphaZero only memorises the move it is going to play, Athénan also retains the simulations generated during the calculations that led to a particular move. As a result, it produces much more data for the same number of matches, which is important considering that a great deal of information is needed in order to learn well." Furthermore, with AlphaZero the value of a state is strongly determined by the end of the game: if it ends in a loss, it will learn that all stages were losing ones, even if the early moves were good, before being followed by bad decisions. With Athénan, a state's value is updated by taking into account such reversals, which allows it to better capitalise on knowledge from one match to another.
Applications in other fields
"To produce good results, all Athénan needs is a 'classical' computer equipped with a graphics card and an average number of processors. AlphaZero, on the other hand, generally needs a supercomputer with a hundred such cards and just as many processors," stresses Cohen-Solal. In short, at least for certain games, Athénan is as good a system as any, while using fewer resources! In the future, the scientist hopes to measure it against a human champion – "or AI that outperformed such an expert" at a particular game such as chess – in order to "achieve strong results which would prove that our software can attain a superhuman level".
Beyond games, the team hopes to test Athénan in other fields that are more practical but also require reflecting on complex solutions. "It could help improve the routing of Internet connections; minimise the number of kilometres driven and that of the vehicles deployed by companies for making rounds (French Postal Service, etc.); and determine the structure ('sequence') of a given RNA molecule based on its shape, in order to boost biomedical research," Cazenave says. For Athénan, it is early days still.
- 1. "Minimax Strikes Back," Q. Cohen-Solal and T. Cazenave, 19 December 2020. arXiv preprint: https://arxiv.org/pdf/2012.10700.pdf
- 2. Laboratoire d'analyse et modélisation de systèmes pour l'aide à la décision (CNRS / Université Paris-Dauphine).
- 3. "Mastering the game of Go with deep neural networks and tree search", D. Silver et al., Nature, 28 January 2016. https://doi.org/10.1038/nature16961
- 4. "Mastering Chess and Shogi by Self-Play with a General Reinforcement Learning Algorithm", D. Silver et al., 5 December 2017. arXiv preprint : https://arxiv.org/abs/1712.01815
- 5. "Best-first minimax search", E. Korf and D. Chickering, Artificial Intelligence, July 1996. DOI 10.1016/0004-3702(95)00096-8
Keywords
Share this article
Author
A freelance science journalist for ten years, Kheira Bettayeb specializes in the fields of medicine, biology, neuroscience, zoology, astronomy, physics and technology. She writes primarily for prominent national (France) magazines.
Comments
Log in, join the CNRS News community