You are here
Computing the Cost of Computation
Buying a supercomputer is no mean feat. Other than the investment itself (approximately €25 million for the first partition of the Jean-Zay machine, already one of the most powerful computers in France, to be followed in late 2020 by a second partition), acquiring a supercomputer is a special process. It is not a matter of test-driving and choosing a vehicle at a car dealer’s. To begin with, most supercomputer vendors are not manufacturers but systems integrators: they buy the main components of the machine (processors, computational accelerators, memory, storage space, parts of the internal network, etc.) from various suppliers, many of whom are the same for all sellers!
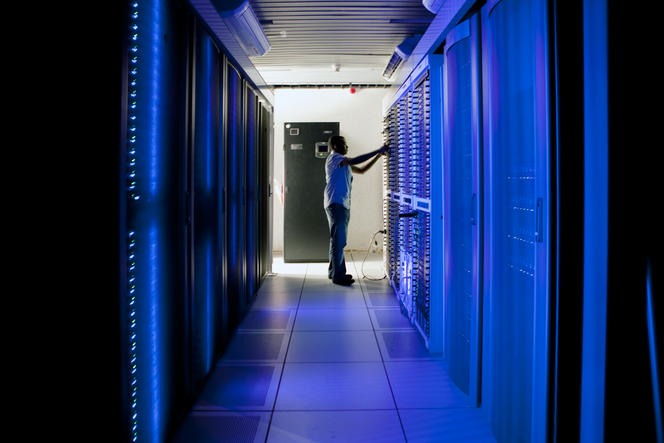
The tight memory market
As a result, supercomputers of different brands can contain the same Intel processors, NVIDIA graphics accelerators, DDN flash storage arrays, Mellanox networks, etc. The difference lies in the quality of the components’ integration into the machine, the physical organisation of the internal network (the speed of communication among the various parts is a key factor in overall performance) and the software environment. The price of a supercomputer, on the other hand, partly depends on the tariffs negotiated by the vendors with their suppliers, which can vary in proportion to the quantities purchased.
One detail is worth noting: the international market for computer memory is very tight, with prices determined by the going rates on the date of delivery to the manufacturer – not on the date of the order. They can rise sharply if several smartphone suppliers place huge orders! Naturally, the contracts include provisions for updating the price or the configuration. In addition, a supercomputer cannot be tried out before buying: there are only a limited number of them on the market, and vendors cannot afford to set aside an exorbitantly expensive test machine for potential purchasers. Even if they wanted to, it would be impossible, as digital technology evolves very rapidly and every customer wants the fastest, most efficient computer possible – which often doesn’t yet exist when the order is placed.
In this case, the purchase agreement for the Jean-Zay machine, financed by the Genci,1 was signed before the Intel Cascade Lake processors that it uses were available. In such a delicate situation, the buyer has to trust the vendor, which in turn must test the customer’s programmes on machines that are not even a scaled-down version of the final product, extrapolating the results in order to promise, under contract, performance levels that are not verifiable but whose breach could lead to penalties. It’s like if a car manufacturer offered to deliver in six months a car that has never been tested, with a contractual guarantee of 30 kilometres per litre!
A novel energy solution
In terms of energy consumption, a supercomputer is a virtual black hole. The Jean-Zay instrument needs a power level of about one megawatt at a cost of more than one million euros per year. This is a huge electric bill, but as computers evolve from one generation to the next, they become faster and also smaller, consuming less energy for comparable computational power – which has made their electrical costs a key selling point. And the machine’s own consumption is not the only consideration: it also must be kept cool (nearly all of the electricity used by the computer is transformed into heat), which typically increases the bill by 20 to 50%.
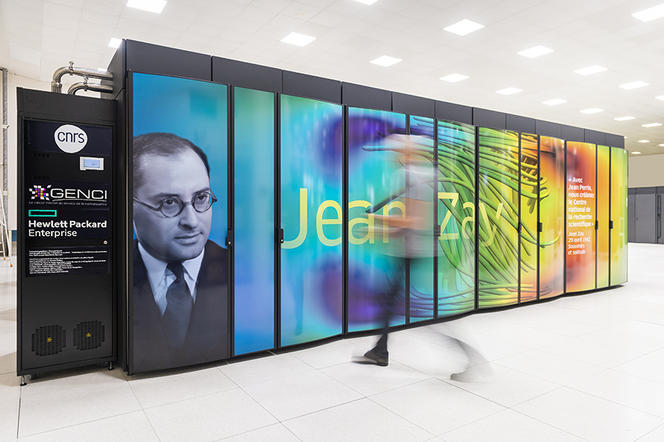
In the case of the Jean-Zay, a different type of cooling system will enable substantial savings. The previous generation of supercomputers used cold water (12°C at the intake), whereas the new processors can withstand higher temperatures and are cooled directly by the circulation of hot water among the circuit boards. The water enters at 32°C and exits the machine at 42°C. Obviously, cooling the water down to 32°C requires less energy than cooling it to 12°C. It’s barely an exaggeration to suggest that the pipes could simply be routed outside the building – at least in wintertime. In addition, this heat will be recovered for heating the campus’s buildings.
A dedicated AI module
What makes the Jean-Zay supercomputer unique is the inclusion of a module dedicated to artificial intelligence research (AI) as part of the “AI for Humanity” plan adopted by French president Emmanuel Macron. This means two things: not only is this machine being made available to a research community that has not previously had regular access to the national large-scale computing centres, but it also incorporates a module specifically adapted to their needs.
Every user wants faster and faster computation speeds. For a long time, this was achieved by improving the performance of the individual processors. This approach is now outdated for two reasons: first, the etching of circuits is approaching the physical limits of silicon-based technologies (disruptive technologies like the quantum computer will not be operational for years to come), and secondly, boosting processor speed drastically increases energy consumption. As a result, other solutions are needed for enhancing a computer’s performance: using multiple processors working together (massive parallel machines), developing vector accelerators that can process a vector all at once rather than a single number, or using other types of accelerators, in particular GPUs (graphic processor units).
AI’s best friend
Unfortunately, the more complex the hardware, the less versatile the machine. For any given problem, certain architectures are better suited than others. In fact, GPUs were not developed for supercomputing, but for video games! They were designed for the rapid processing of images – hence the link with artificial intelligence, or to be more precise with machine learning, one of whose goals is to enable a computer to identify an object in an image: a cat, a cancerous tumour, etc.
As a machine analyses more and more images, it perfects its “skill” to the point of being able to recognise the designated object with great accuracy. This is the advantage of artificial intelligence in medical imaging: a specialist doctor hones this ability by examining no more than a few dozen x-rays every day, whereas the machine can learn from tens or hundreds of thousands of images, given sufficient processing capacity. From an IT point of view, a collection of images is only a large database, so the techniques initially developed for imaging can be used in other fields. GPUs are especially well-suited for processing massive amounts of the type of data needed for machine learning, no matter what the intended application. At first, the Jean-Zay machine will incorporate 1044 GPUs, a far cry from the handful of such units that currently equip most AI researchers’ workstations, thus offering them many new possibilities. And, of course, the GPUs can also be used by researchers in other disciplines who need that kind of computing power.
The points of view, opinions, and analyses published in this column are solely those of the author. They do not represent any position whatsoever taken by the CNRS.
- 1. Grand Equipement National de Calcul Intensif, a non-commercial corporation founded in 2007. The French government, through the Ministry of Higher Education and Research, owns 49% of the capital. The CNRS and CEA each hold 20%, while 10% is held by the French university network and 1% by the French National Research Institute for the Digital Sciences (Inria).