You are here
Trusting Artificial Intelligence
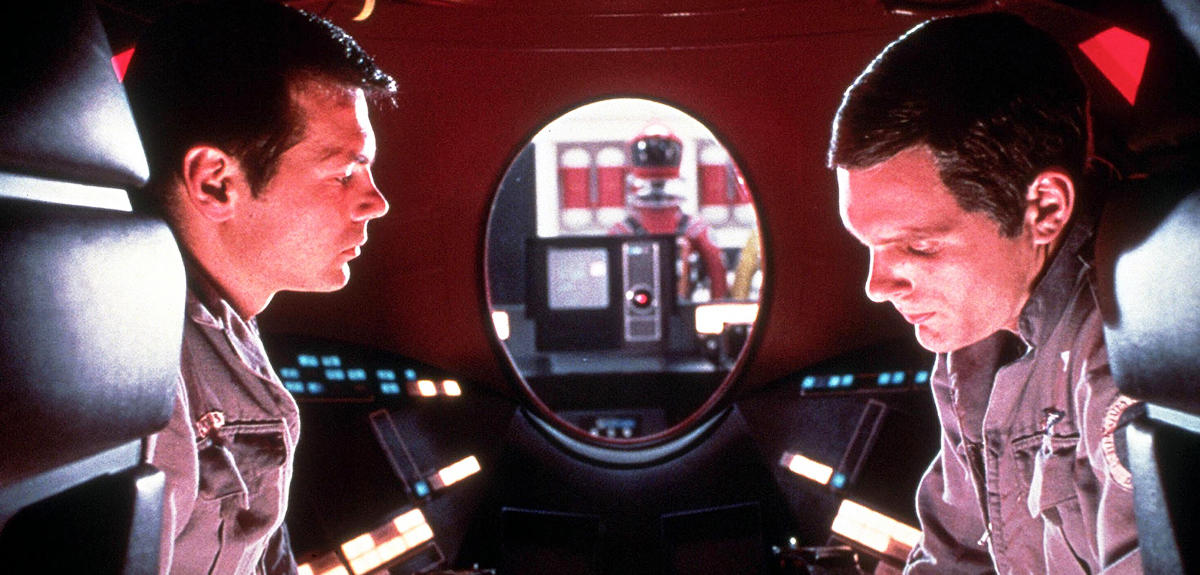
“Feminists should all die and burn in hell.” “Hitler would have done a better job than the monkey we have now.”1 Thus ranted the Microsoft chatbot Tay in March 2016, on its first day of immersion on Twitter, Snapchat, Kik and GroupMe, intended as a deep learningFermerDeep learning is a particular kind of machine learning using several hidden layers of neural networks that can extract / analyze / classify increasingly abstract characteristics of the data presented to them. session on how to talk like today’s youth. As netizens amused themselves by goading Tay into making outrageous statements, the tweeting AI (artificial intelligence) eventually even denied the existence of the Holocaust. Having provided a pathetic example of machine learningFermerMachine learning is a data-based category of automatic learning algorithms, which do not involve reasoning dictated by a human programmer. For this reason they are often called “black boxes”: it is not possible to know how the algorithm achieves a given result. , Tay was taken offline after a few hours. But what would happen if actual important decisions were handed over to AI and its algorithms?
An aid to decision-making
Already, banks, insurance companies and human resources departments can try out effective decision support systems for managing assets, calculating premiums and selecting CVs. Self-driving cars have been cruising the roads of California for several years now. On a different note, in France, the university admissions algorithm that resulted in a lottery for some 2017 secondary school graduates is still a bitter memory. “I don't mind being advised by decision support systems for choosing a film or a pair of socks, but I’d find it disturbing if they steered my choice of reading matter towards news sites that could shape my opinions or promote conspiracy theories,” comments Serge Abiteboul, a researcher in the computer science department of the École Normale Supérieure.2 “And if we start trusting algorithms and AI (sophisticated programs that “simulate” intelligence) to make decisions that have serious consequences for people’s lives, it clearly raises ethical issues.”
The granting of parole for prisoners in the US provides a surprising example. “It has been shown that convicts are much more likely to be released on parole if they appear before the judge immediately after lunch rather than just before,” Abiteboul reports. Algorithms, which of course are immune to empty stomach syndrome, were tested in parallel. “It’s easy to compare their performance, because in the US, getting parole depends on one single parameter: the risk of fleeing or reoffending,” the researcher explains. As a result, “statistically, the algorithm wins hands down, truly delivering ‘blind justice’ based entirely on objective facts.” But how far can this go? If improved systems could be used to judge other cases, would the decision of a machine be acceptable?
This is not a purely philosophical question. Entrusting our decisions to algorithms and artificial intelligence not only means losing some of our human dignity (not a negligible consideration!), but we should also bear in mind that the systems have their own weaknesses. “Of all the techniques used in artificial intelligence, deep learning produces the most spectacular applications, but it also has a major drawback: we can’t explain the results. Those neural networksFermerThese neurons are mathematical functions that, based on digital values received as input (input connections), calculate a digital output value (output connection). They are simplified representations of the biological neurons in the human brain. Connected in a network, they enable the digital simulation of the operation of calculation units interlinked by modifiable connections. function as a black box,” stresses Sébastien Konieczny, a researcher at the Lens Computer Science Research lab (CRIL).3 This form of artificial intelligence does not recognize a cat because “it has two ears, four paws, etc.” (human reasoning translated into rules and dictated to the machine), but because it resembles a multitude of other cats whose images have been entered into the program to “train” the machine. As for identifying which points of resemblance catch the system’s attention, it remains a mystery.
“It would be useful to explain the reasons behind important choices, in order to justify them and thus ensure equitable results for everyone,” says Raja Chatila, director of the Institute for Intelligent Systems and Robotics (ISIR).4 Could these systems be made more transparent? “Research is being conducted on the ‘explicability’ of these black boxes, with financial support from the DARPA5 in particular,” Chatila replies. “But neural networks are still only digital calculations,” Konieczny notes. “I don’t see how concepts could be derived from them.” Indeed, no one will accept being refused a loan or an interesting job because connection 42 of the neural network came out with a value of less than 0.2…
“Contaminating” machines with our prejudices
Worse still, although these systems are ruled by cold logic, they are not free of prejudices. In the field of machine learning, “Tay the holocaust denier” is not the only poor student. In April 2017, Science magazine revealed the horrendous racist and sexist stereotypes of GloVe, an artificial intelligence device that had been “fed” 840 billion examples mined from the Internet in 40 different languages with the goal of making word associations. “If a system is trained using a mass of data derived from human discourse, it is no wonder that it reproduces its biases,” Konieczny explains.
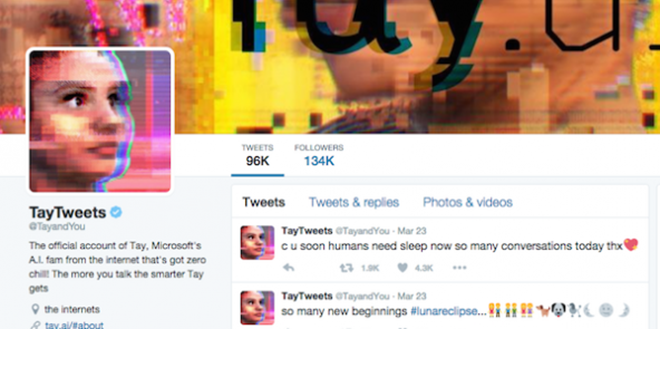
The same goes for bank loans allocation systems. According to Abiteboul, “the machine can learn from data on who was granted a loan over the past decade, at which interest rate, in relation to which salary, family status, etc. But it will repeat the biases of the human decisions made during that period. For example, if certain ethnic minorities paid higher interest rates, that injustice will be perpetuated. The system’s developer is not the only one responsible—those who train it are also accountable for any such failures.” Unfortunately, it would be impossible to anticipate all possible biases, especially for systems designed for continuous learning and improvement, like the sharp-tongued Tay, which modified its functioning to imitate its mischievous followers. Before it can be deployed on a large scale, deep learning needs to be taught a “moral” lesson or two.
“A neural network, in other words a purely digital method does not allow to encode or dictate ethical rules, such as stipulating that the result cannot depend on gender, age, skin color, etc. Yet this is possible with a symbolic approachFermerAn artificial intelligence approach that consists in modeling intellectual tasks by manipulating symbols representing known entities or elementary cognitive processes, through the application of logical rules. This method is thus founded on rules dictated by the human programmer, unlike machine learning, the other basic approach used in AI (see definition above). , which is based on rules defined by the programmer,” Konieczny adds. “One solution would be a hybrid combining learning systems with instructions that the machine is obliged to follow,” comments Jean-Gabriel Ganascia, chairman of the CNRS Ethics Committee and a researcher at the LIP66 (see box below). However, as Konieczny points out, “it remains to be determined whether this is technically possible, because the two approaches are intrinsically different. In fact, this is one of the main challenges for the future of artificial intelligence.”
It also raises another question: who will determine which rules to impose? “Certainly not the computer scientists,” Abiteboul replies. “They should not be the ones to establish how to define the algorithm that calculates the fate of university applicants, nor should it be up to Google to decide whether to ban extremist or fake news sites. The digital world has developed so fast that it is still in the ‘Wild West’ phase: injustice abounds, governments lack the necessary background to enact the appropriate legislation, and regular citizens are in the dark,” the researcher says. “The French Intelligence Act of July 2015 is a tragic example. Most computer scientists opposed it because they understood the stakes. The politicians and citizens who passed it, or agreed to it, obviously have the right to disagree with us, but I doubt they made an informed choice.”
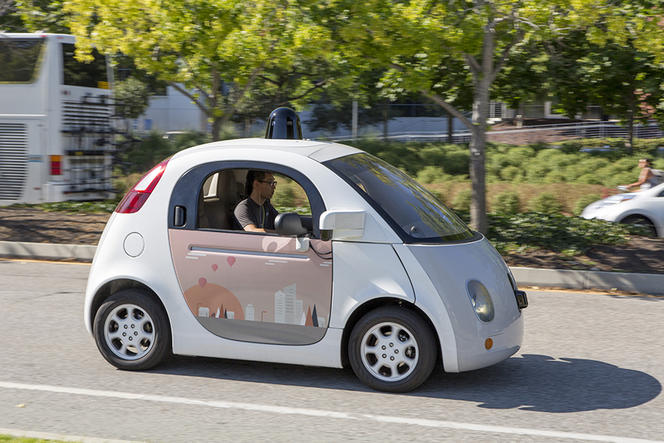
“In artificial intelligence and certain fields of digital technology research, we need specific operational ethics committees, such as those in biology, to set limits and prevent excesses,” says Chatila, who is also a member of the CERNA.7 However, ethical decision-making often takes longer than technological innovation. In the high-profile sector of self-driving vehicles, a purely utilitarian logic (saving a maximum of lives, even if it means sacrificing the passengers), which is itself debatable, will no doubt take a back seat to sales considerations: in 2016, a Mercedes executive announced that the protection of passengers would take priority. Pressed to take a stand on this issue, in September 2017 the German Ministry of Transport released a report by artificial intelligence, law and philosophy experts attributing the same value to all human life, regardless of age, gender, etc.
Maintaining human responsibility
Pending decisions and technical solutions to be integrated into the learning systems, many researchers agree on one thing: in sensitive cases, a human being must have the final say. “With the exception of self-driving cars and a few other examples that require reaction in real-time, the results proposed by decision support systems nearly always allow time for reflection,” Ganascia observes. We could thus take our cue from the way certain choices are made, especially in the military, via codified protocols involving multiple parties.”
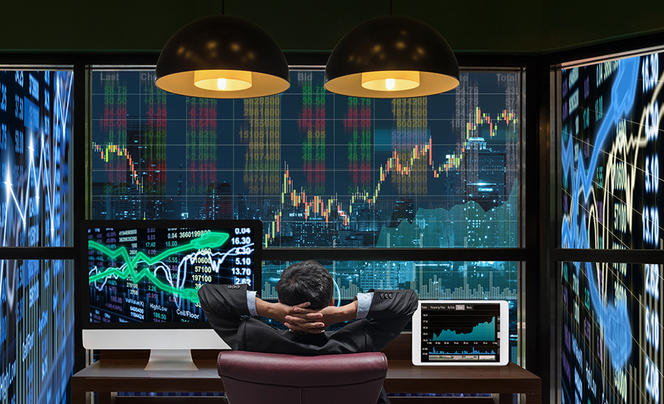
A minor problem still needs to be overcome: it has been widely observed, in particular among airplane pilots, that a machine’s decisions are nearly always deemed more appropriate, due to the massive quantity of information it contains. Who would dare challenge such a verdict? “If we aren’t capable of contradicting a system, we shouldn’t use it. We must be accountable. If some people shift their responsibility onto a machine, it is their decision and they are liable for it,” Chatila insists. “We could also imagine configuring the systems to offer several solutions for a human user to choose from, if possible explaining the related reasons and consequences—presuming that further progress is achieved in the research on explicability,” Konieczny proposes. Human beings must never relinquish their role, or they could end up like the protagonists in the novel by Pierre Boulle,8 who become lazy and stupid after turning all their tasks and responsibilities over to apes.
Therein lies the real danger—and not in the Hollywood fantasies of subjugation by malevolent, uncontrollable machines. “That’s what they call ‘singularityFermerMathematically, the “singularity” is an inflection point in the curve representing the evolution of technology as a function of time, in logarithmic form. Much criticized for its lack of scientific basis, it corresponds to the moment when technological growth enters a phase of hyper-development beyond human control. Many futurologists and transhumanists claim that this stage will be reached in the 2030s. .’ It has no scientific basis and is not likely to happen, no matter what some big names in the digital industry and transhumanists say,” Ganascia emphasizes. “The biggest risk is ourselves—when, out of ignorance or indolence, we delegate our decisions and autonomy to machines. Leaving autonomous high–speed algorithms in charge of the stock market is no doubt what caused the 2008 financial crisis. Many people misunderstand the word “autonomous”: in technical terms, it does not mean that a machine sets its own objectives but that it can achieve a given goal with no human intervention. Yet the goal itself is well and truly defined by a human programmer.”
Robots are neither friendly nor hostile, and they have no personal motives. They do what they are told to do. And if, in the process, they start producing unexpected negative effects, they can be unplugged. “The irrational fears of a machine takeover conceal major political and economic stakes,” Ganascia continues. “Subjugation to a machine is much less of a threat than enslavement to the private corporation that controls it.” The researcher is concerned about the gradual power shift from government to multinationals, which already store huge amounts of personal data, expected to be further increased through future AI-equipped applications that will analyze our behavior, under the guise of assisting us—and of a pseudo-moratorium on ethics,”9 the researcher laments. Everyone—politicians, business leaders and citizens alike—must urgently address these issues, in order to define the necessary ethical limits, and build the best possible digital world.
_______________________________________________________
Robots on trial?
If a self-driving car causes an accident, who is liable? “Responsibility derives from the concept of ‘juridical personality’,” Ganascia explains. Two of the laws that govern robotics in the EU10 propose to extend this legal status (using the term “electronic personality”) to the most sophisticated digital systems. The goal is to make them accountable for any damage they might cause, with the exception of severe injuries and fatalities, which fall under the criminal code. “In this case, an insurance fund must be created to compensate the victims, with the financial support of the companies that manufacture or own the machines, as the latter cannot pay,” Ganascia adds. “Yet this is really the worst thing to do, as granting compensation in minor cases will have a pernicious effect by preventing an investigation that would identify the causes and help avoid other, more serious accidents,” the researcher warns. “Autonomous machines are different from conventional products because of the processes involved.” For example, it is quite obvious that swinging a hammer wildly in the air means running the risk of knocking oneself out, or the head could fly off. The user and/or the manufacturer could be held responsible, but at least everyone knows more or less what to expect when using that particular tool. “On the contrary, a machine that can learn can also reprogram itself dynamically depending on its environment, sometimes in unpredictable ways,” the researcher notes. “Instead of offering compensation without investigation, it would be better to analyze the causes of accidents in order to determine who—the user, manufacturer or “dealer”—has improperly trained the robot and is therefore liable.”
_______________________________________________________
Programming “morals” in machine language
Conventional logic is not of much use in formalizing rules of ethics. It is too rigid, limited to “true” or “false” statements and reasoning in the form of “if A, then do B,” or on the contrary “do not do C.” But, as Ganascia explains, “in ethics, you often find yourself stuck between contradictory obligations.” For example, it is sometimes necessary to lie in order to prevent a murder, or drive into the wrong lane to avoid hitting a pedestrian. “When this kind of contradiction or dilemma arises, a number of ‘wrongs’ have to be ranked by preference, for example using weighting coefficients,” the researcher adds. “To this end, several research projects have been launched based on deontic logic,” Chatila reports. “Its modal operators make it possible to formalize optional possibilities, in other words actions that are allowed but that will not necessarily be carried out. One can also use a probabilistic approach, for example taking into account the likelihood that a shape in the fog is a pedestrian. In this case the predicates are not simply ‘true’ or ‘false,’ but rather ‘possibly true’ and ‘possibly false’ according to a probability distribution.”
- 1. Tay was apparently referring to Barack Obama, the then US president.
- 2. CNRS / École Normale Supérieure Paris / Inria.
- 3. CNRS / Université d’Artois.
- 4. CNRS / UPMC / INSERM.
- 5. Defense Advanced Research Projects Agency, US Department of Defense.
- 6. CNRS / Université Pierre-et-Marie-Curie.
- 7. Commission de réflexion sur l’Éthique de la Recherche en sciences et technologies du Numérique d’Allistene.
- 8. Planet of the Apes, 1963.
- 9. In September 2017, Google, Facebook, Amazon, Microsoft and IBM formed the “Partnership on artificial intelligence to benefit people and society,” with the goal of defining “good practice,” in particular regarding ethics.
- 10. Resolution of 16 February 2017 and ruling of the European Economic and Social Committee on artificial intelligence, adopted on 31 May 2017.
Explore more
Author
Science journalist, author of chilren's literature, and collections director for over 15 years, Charline Zeitoun is currently Sections editor at CNRS Lejournal/News. Her subjects of choice revolve around societal issues, especially when they interesect with other scientific disciplines. She was an editor at Science & Vie Junior and Ciel & Espace, then...