You are here
Takes Two: Why We Learn Better Together
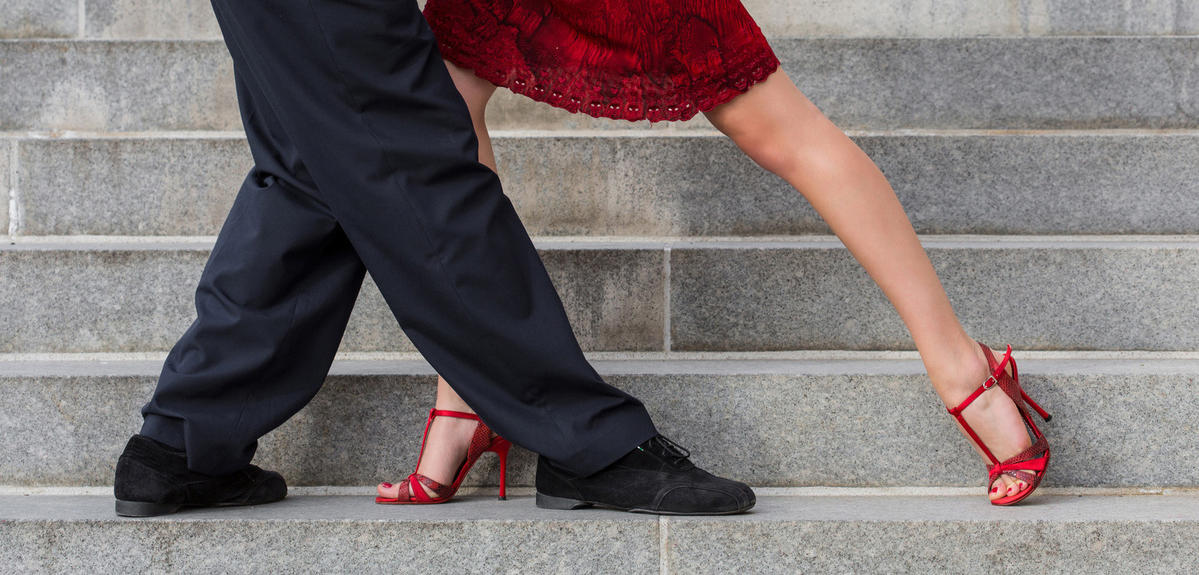
Whether it’s a case of a parent guiding a toddler’s first steps by hand or a tango teacher running a pupil through dance steps, humans develop their motor skills by interacting with one another, hands-on.
Scientists have already established that duos can enhance their execution of tasks via the tactile exchange of information between them. Yet they were unsure of the nature of the information they shared. Now, by combining computer simulations and empirical tests, a team of neuroscientists and roboticists has pinpointed how twosomes egg one another on by guessing at the goals of their partner’s movement.1 Building on their find, the team was able to develop an algorithm reproducing this type of human behavior, which in turn promises to improve human-robot interactions like those used in manufacturing, powered exoskeletons or physical rehabilitation.
They say that two heads are better than one. And when it comes to haptics—the study of sensory data transmitted by touch and proprioceptionFermerAn ability to sense the position of one’s body parts without visual cues, used for example, to touch one’s nose with eyes closed.—the same goes for bodies. Ganesh Gowrishankar from the JRL2 in Japan, along with colleagues from Imperial College London (UK) and the Advanced Telecommunications Research Institute (Japan), had previously experimentally demonstrated that pairs of individuals boost their motor skills through physical interaction with one another.3
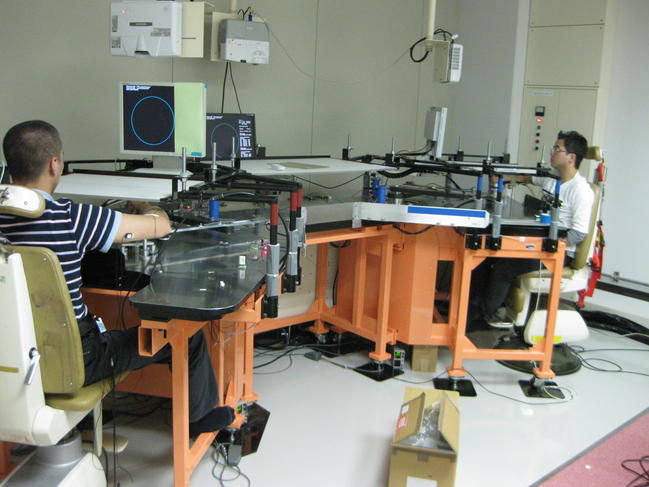
A virtual elastic band
In their setup, human subjects were asked to track for an hour a randomly-moving target on a computer screen by moving a cursor—in this case a hand-operated robotic device, rather than the classic mouse. Individuals operating alone turned out to do less well than when—unbeknownst to them—they were linked to a partner by what Gowrishankar describes as a “virtual elastic band,” giving them a sense of their unseen partner’s movements. Surprisingly, this earlier study also showed that physical interaction improves the performance of both partners, regardless of their respective skill levels: “Even when individuals had partners that were worse than them, this type of ‘contact’ still helped them improve their performance.” Which prompted Gowrishankar and his colleagues to ask one crucial question: “What exactly is the information transferred between a pair that helps both partners improve their skills?”
To test out different hypotheses on the nature of this information, the researchers’ new study modeled the outcomes of four different scenarios, before comparing them with the first study’s results. The first three models reflected pre-existing theories on how partners react when working together: one without any exchange of haptic information, another where they follow the partner when they feel the other is performing better, and the third where they combine haptic feedback on their own position with estimates on their partner’s position.
But it was the fourth model, formulated by the researchers themselves, that proved the most closely aligned with the experimental data. Their simulation showed what happens when the individual uses haptic feedback to infer the goal of the partner’s movement. “We assume that a person models their partner’s behavior on his or her own behavior,” explains the JRL researcher. “So when the person senses their partner’s movement, he or she will infer the goal by guessing where this movement may lead.” An inference that allows individuals to sharpen their own perception. “By estimating your partner’s prediction on where the target is going, you can improve your own target estimation, and hence your performance.”
Can you feel the robot?
The discovery that individuals guess the intended objective of their partner’s movement to perfect their own performance left the researchers with one major query: could the coupling of a human with a robot yield the same performance-improvement? The answer was unequivocal: further experiments matching individuals with a robot running a team-designed algorithm that mimics human haptic responses, indicated that humans consistently hone their skills with the robot, whether the latter displays superior, similar or inferior motor skills.
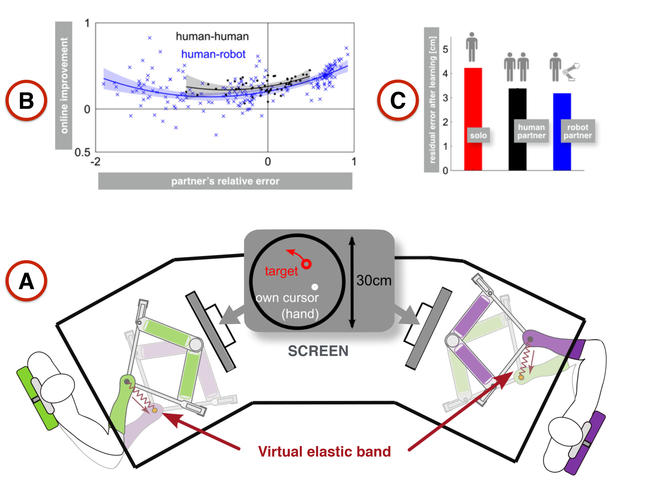
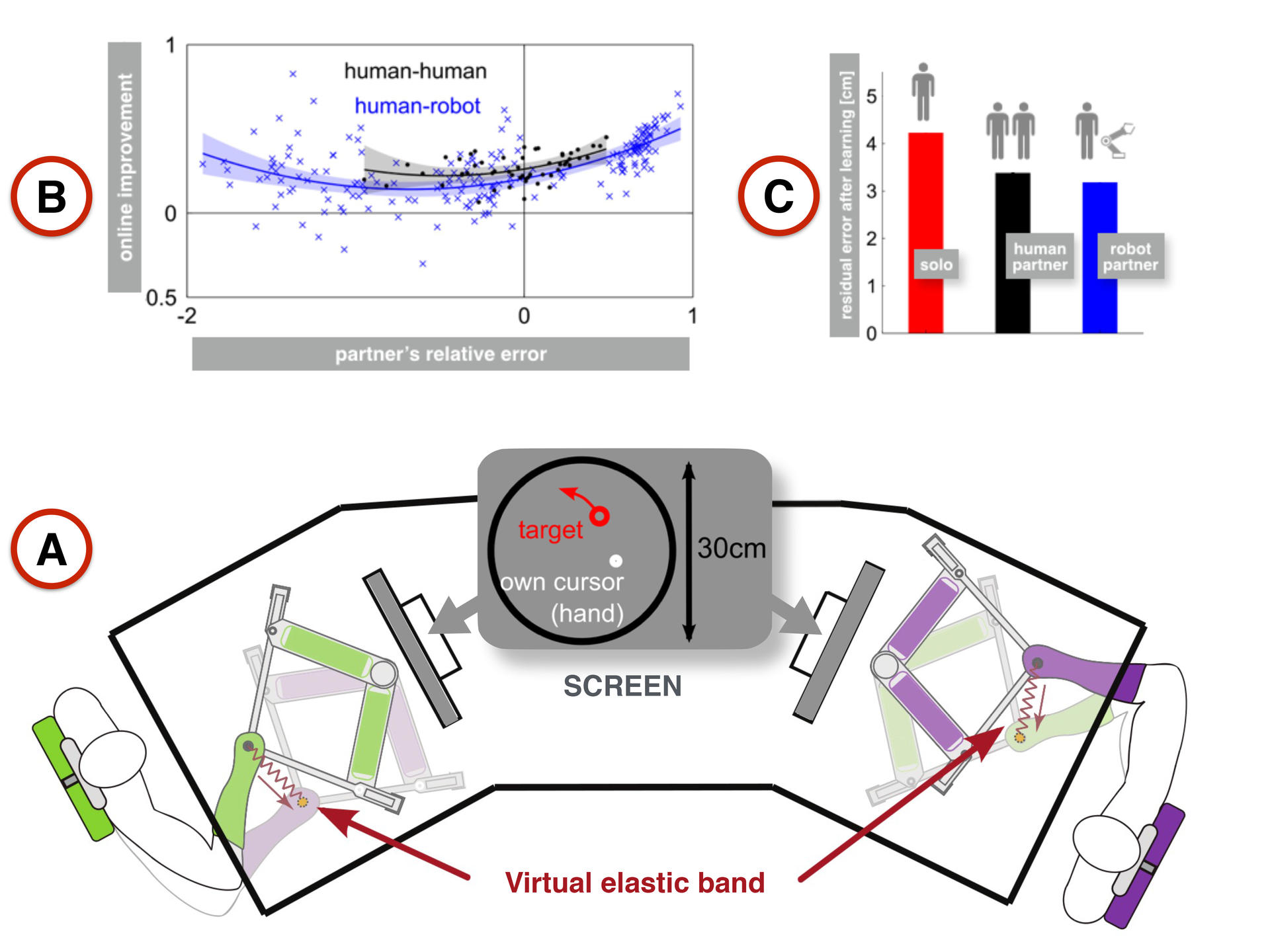
From exoskeletons to rehabilitation
Importantly, by producing an algorithm that induces humans to fine-tune their skills, the team paves the way for new human-centered robotics applications in manufacturing, for example. “A current industrial trend is having robots that work directly with humans, as seen in the car manufacturing industry. These algorithms could help us develop applications that optimize these interactions,” suggests Gowrishankar. Interactions namely seen in assembly, transport or loading operations, where human input visual or tactile skills while robots contribute force.
Alternatively, the algorithm can enhance the behavior of powered exoskeletons—technology-boosted suits which amplify the wearer’s strength and endurance, used for example in heavy-duty military or rescue operations. A core issue raised by these outfits, specifies Gowrishankar, is the sharing of control between robot and human: “We can’t have the human controlling everything, otherwise why have a robot at all? At the same time, robots of course can’t take full control. So we need a system where both carry out the task and compensate for the other’s mistakes without interfering with one another.” According to the researcher, the team’s interaction-based algorithm takes “an important step in this direction.”
The algorithm could also have a future in rehabilitation robotics, where robots act as exercise-therapy aids that can help people regain certain motor skills following illness or injury. To probe the algorithm’s potential in this domain, the team aims to set up collaborations with rehabilitation hospitals. “Rehab patients suffer from a wide range of diseases and conditions, and we have to narrow down the categories of sufferers for which the application could be used,” reports the roboticist.
In the meantime, the team continues to deepen their understanding of the pair-interaction phenomenon, namely the neuroscience at work. “Estimating a partner’s target from their actions is a form of mind reading known as ‘action understanding,’” notes Gowrishankar. “How far does this type of understanding go? Can we even understand a partner’s intentions, emotions and thoughts from haptics?” Further studies may provide answers.
- 1. A. Takagi et al., “Physically interacting individuals estimate the partner’s goal to enhance their movements,” Nature Human Behaviour, 2017. X: 0054, DOI: 10.1038/s41562-016-0054.
- 2. Joint Robotics Laboratory (CNRS / National Institute of Advanced Industrial Science and Technology).
- 3. G. Gowrishankar et al., “Two is better than one: Physical interactions improve motor performance in humans,” Scientific Reports, 2014. 4: 3824, DOI: 10.1038/srep03824.
Keywords
Share this article
Author
As well as contributing to the CNRSNews, Fui Lee Luk is a freelance translator for various publishing houses and websites. She has a PhD in French literature (Paris III / University of Sydney).
Comments
Log in, join the CNRS News community