You are here
Will the Androids from the Alien Saga Exist in 2093?
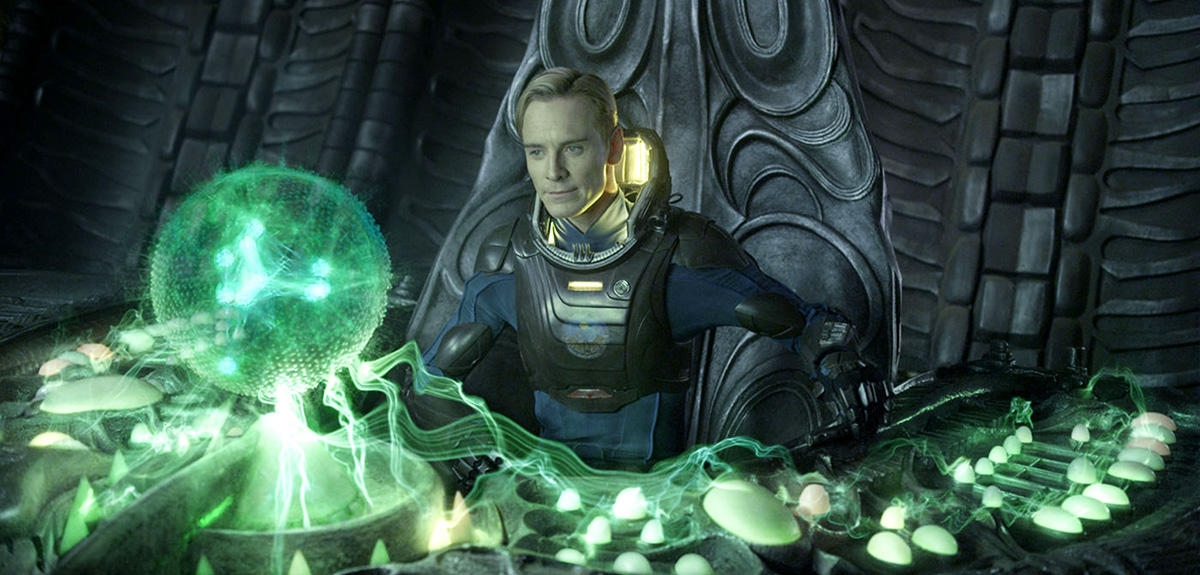
Warning, this article includes spoilers.
The parasite monster of the title role tends to obscure the androidsFermerRobot that resembles a human. Etymologically it means "that which resembles man," (from the Greek andros, "man"). The term "humanoid" is also used. , which are everywhere in the Alien saga, as emphasized in your book published a few days ago. Can you remind us of which robots are involved?
Frédéric Landragin:1 The first one, by order of appearance, is Ash, the android from Alien (Ridley Scott, 1979). He could pass as human so well that his artificial nature is only discovered during the last third of the film. Then there is Bishop, who is less impressive and less present in Aliens (James Cameron, 1986) and Alien 3 (David Fincher, 1992). Then there is the unforgettable Call in Alien: Resurrection (Jean-Pierre Jeunet, 1997), who looks more human and compassionate than Ripley, who has of course become a hybridized clone with alien DNA. Finally, there is the Machiavellian David in Prometheus (Ridley Scott, 2012), and his rival Walter in Alien: Covenant (Ridley Scott, 2017). Of course, creating a machine with emotions and personal goals that can pass for human, like those on this list, is totally fanciful today.
The perfection of their appearance and their movements (Call is so agile she plays at grabbing a cup with boxing gloves!) also seems a far cry from what is being produced in the laboratory or industry. But what if we limit ourselves to just language and its understanding.
F.L.: Even in that case, we're still wide of the mark! In Alien Ash speaks fluidly, participates in conversations, and puts forward his opinions and arguments: no real robot can achieve such a level of language mastery. And consider "Mother," the neutral and factual on-board supercomputer who is not embodied, but who takes questions from the crew of the Nostromo through a keyboard: despite her vast limitations compared to the android Ash, she far surpasses the capacities of the talking machines of 2019. Mother speaks and understands human language, so you can formulate requests without writing a program in computer language, or thinking about a specific way of communicating.

But this is already what we do with the assistants Siri or Cortana, or when we tell Alexa to play a song, right?
F.L.: Siri and Cortana are just interfaces toward search engines, and don't understand the first thing about anything. Like Alexa, the companion robots Nao and Pepper, all of our talking machines function with keywords, and have no access to the meaning of sentences, which is to say to semantics. This is the rub in Natural language processingFermera field that provides machines with language, whether it is for translating, summarizing, or conversing fluidly with an artificial intelligence. It notably consists of modelizing language in abstract (mathematical) representations, and brings together linguists and computer scientists. (NLP). Especially when it comes to correctly interpreting a word in relation to its neighbours and other sentences, and avoiding ambiguities in languageFermerAmbiguity in language: for example, "the pen is in the boxla pièce est dans le porte-monnaie" (the coin is in the purse) and "the box is in the penle porte-monnaie est dans la pièce" (the purse is in the room) contain the same words with the same syntactical relations, but the meaning of the word "piècepen" is different: in the first case it’s a pièce de monnaie (a coin)writing instrument, and in the second it’s an enclosurea room in an apartment.. A talking system must also use what it "knows" about our world, through an ontologyFermerIn artificial intelligence, this involves sums of information and concepts that can describe anything. Some are highly advanced but limited to very specialized domains, for example to very precisely describe planes, from the seats to the control levers all the way down to the clasps that hold cups in place. They are used to quickly find very specific information in a large technical manual. An ontology to describe our entire world would be extremely costly. that describes it.
But an ontology describing our entire world would surely be impracticable.
F.L.: Exactly. Alexa and the others manage because the vocabulary and sentences for volume settings and song choices are very limited, and therefore relatively easy to describe exhaustively. But discussing any subject—even in a documentary and emotionless way like Mother—is a whole other ballgame! Our machines are incapable of this, and even less so of the irony used by Ash and David. Common sense, irony, comebacks, etc., are all shaped by what we experience and feel when we interact with our peers and the environment through our bodies. All of this would have to be reproduced in order for a robot to demonstrate this.
And what if machines lived with us so they can learn from our conversations through machine learningFermerA class of automatic learning algorithms based on data, with no explicit production of rules. In this kind of artificial intelligence, a cat isn't recognized because "it has two ears, four paws, etc." (human reasoning consisting of rules and dictated to the machine), but because it resembles a large quantity of other images of cats provided for the machine in order to "train" it. We ultimately don't know what resemblances make it click! That's why these algorithms are referred to as "black boxes." (one of the approaches used in artificial intelligence)? In Prometheus, we see David repeating comebacks from other films.
F.L.: There have been prototypes that learned in this way—on their own and on the go—based on conversations picked up in their environment, but they only learned a billionth of what we wanted! Look at the disastrous results by Tay, the Microsoft chatbot that was disconnected in 2017 after just a few hours of functioning, due to its proven racism and sexism. Instead of letting the machine learn on the go, we instead do supervised learning, by providing them with carefully chosen examples.

These learning techniques based on examples, especially deep learningFermerMachine learning technique that uses neural networks (mathematical functions). They are capable of extracting, analysing, and classifying abstract characteristics from the data presented to them, with no explicit production of rules. We don't know why the system arrives at its results, it's a "black box.", have yielded spectacular results in recent years in the field of image recognition. Can they not also enable the acquisition of language?
F.L.: Yes, but a machine learns less efficiently than a human. A child can very quickly detect the interesting points, regularities, exceptions, etc. It only takes two or three examples for them to unconsciously take them into account, whereas about a thousand times as many are needed for a machine to learn something resembling good sense, and to maintain the illusion for any subject of conversation. For a machine to recognize a cat, it has to be provided with thousands of examples indicating that it's a cat (Editor's note: it can find resemblances between the images on the scale of the pixel, but we don’t know which ones: these systems are "black boxes"). Amassing a body of examples of conversations is much more complex.
Why is it so complex to feed a machine with examples of conversations?
F.L.: Because the thousands and thousands of sentences have to be annotated with linguistic information. Because you have to indicate the syntaxFermerRules, especially of grammar, that connect the words of a sentence to one another.: where is the verb, the subject, the direct object, etc., thereby enabling the machine to learn how to identify them (similarly, to acquire irony, thousands and thousands of conversations in which it appears must be emphasized). We have twenty years of such annotations produced by hand, and today we can even generate them with automated systems. But for semantics, it's still humans that are stuck doing the work. They have to specify the appropriate meaning in instances of polysemyFermerPresence of a number of meanings for the same word. For instance, machines struggle with very commonly used words such as "petit" (petit de taille small size, petit bourgeois , petit caractère , etc.) or "jeu" (jeu de clés , jeu vidéo , etc.). All of these nuances of meaning have to be pointed out.], the proper interpretation in cases of ambiguity; indicate who is carrying out the action (an agent), and who is undergoing it (a patient). They also have to annotate the "acts of dialogue acts": whether it's an order that we expect to be executed, or a question that we expect to be answered, or an assertion that we expect to be taken considered as part of a possible reaction (Editor's note: there are examples of this in the opening scene from Alien: Covenant, which shows David interacting with his creator, Peter Weyland).
So deep learning is not necessarily the most promising approach for making machines speak like humans?
F.L.: You are correct. In image recognition, deep learning sparked such a revolution that we will most certainly not return to the symbolic approachFermerAn artificial intelligence (AI) process that uses logic rules. It is therefore based on rules dictated by human programmers, as opposed to machine learning, which is the other major approach used in AI., which consists of rules written by a human programmer. But for NLP, the progress has not been obvious. That is because the image is no more than a matrix of pixels in which repeating forms are identified. It's very formal. Language, on the other hand, raises numerous problems relating to ambiguity, subtext, polysemy, etc. For these tasks connected to the deep meaning of sentences, the symbolic approach is still justified. Another reason for this is possibly related to Zipf's law, which states that a language's most frequently used word is used twice as often as the second most frequent word, which is itself twice as frequent as the third, and so forth. As a result, many words are used very little, and a learning system will encounter them too rarely in the examples provided in order to correctly learn the "behaviour." To improve results, the size of the learning corpus must be multiplied by a hundred or a thousand, which quickly becomes unmanageable.
What kind of subtext is complicated to teach to a machine?
F.L.: Take, for instance, the sentence: "Jean John stopped teasing his wife." A machine would understand that someone named Jean John stopped teasing his wife, and that is all, whereas a human would immediately deduce that Jean John is married, and that at some point he was teasing his wife, which the words do not explicitly say. Machines are very bad at identifying this deeper meaning, even with deep learning. It's to attack this phenomenon that the symbolic approach remains relevant, or rather that people are envisioning systems that combine the two approaches by using the advantages of each one. That's very clearly the future for NLP.
Really? Many researchers in artificial intelligence were telling us two years ago that they didn't know if combining the symbolic approach and machine learning were even possible.
F.L.: People have started doing it in NLP! For example, we can work on texts with linguistic rules (in other words a symbolic approach), before initiating learning. Take the sentence: "the pram doesn't fit in the suitcase because it is too big, so I'll put it somewhere else." "The pram," "it," and "it" indicate the same object, and form a coreference chain. In my laboratory, we are trying to teach machines to identify them. And it's not just a superfluous question, because if I replace "big" with "small," "it" is no longer the pram, but the suitcase! With this variant, the two sentences make up a Winograd schemaFermerGroup of sentences that differ by only one seemingly trivial word, but that have very different meanings. For example, "the alien doesn't fit through the air duct because it is too big," and "the alien doesn't fit through the air duct because it is too small." The resolution of this test notably relies on both lexical knowledge (what an alien and an air duct are) and pragmatic knowledge (like the relative size of a "typical" alien and a traditional air duct). To solve all Winograd schemas, one would have to modelize the complete functioning of our physical world! that the best talking machines can solve only 60% of the time (which is hardly better than choosing a solution by flipping a coin).
So we provide our systems with thousands of examples in which the "its" have been annotated. We hope they will then be able to identify the coreference chains of any text. The problem is that we also say "it is raining," "it is windy," etc. All of these "its" do not at all indicate objects or people, and should not be identified by the machine as belonging to the coreference chain. It is easy to produce a rules-based system to exclude particular verbs. So we are imposing a filter on the text before we initiate learning.

But the two methods are not really mixed. Is there not another way of combining them more "deeply"?
F.L.: There is. It consists firstly of initiating learning on the text, and then analysing the errors to identify their causes. Then we try to correct them by "forcing" the system to give more or less consideration to the parameters that interest us. Injecting a complete thesaurus into the system can indirectly force it to take this linguistic knowledge into account, which has already been formalized as rules. The ideal model would no doubt use machine learning for the most common phenomena, and then direct more rare phenomena toward the symbolic approach, whose rules we will write.
Let's project about 70 years into the future, which takes us to the year 2093, when the story of Prometheus, the prequel to Alien, begins. Do you think an android will be able to speak like David at that time?
F.L.: I have absolutely no idea! The dazzling progress of deep learning at the game of go and in artificial vision surprised the entire world in 2015, so how to foresee what will happen? In the years to come, there will be increasing research on semantics, disambiguation, the management of polysemy, etc. We need at least another ten years to arrive at a fairly well performing system that can replace today's rudimentary systems operate through keywords, and to thereby justify its integration within companion robots, for example. That's about all I can say.
The image of scientists in the Alien saga is nightmarish. In Alien: Resurrection, they clone Ripley after her suicide and use humans, who are bought as "medical material," to implant xenomorphs. Not to mention the mystical archaeologists of Prometheus.
F.L.: You forgot to mention David, who is every bit the mad scientist! Created by humans, androids get mixed up in "creating" in their turn, testing different types of incubation for aliens, and doing so randomly with no research protocol (let alone ethics). Alien is a kind of anti-2001: A Space Odyssey (Stanley Kubrick, 1968), a film that showed scientists who were more realistic, and so serious that they behaved almost like robots.
As a researcher, I prefer Kubrick's vision, because it says more about research (at least that of the period), the feasibility of a journey into space, and the development of artificial intelligence. But Alien is interesting in other respects, for instance showing what explosive things can happen between six or seven people locked up in a spaceship! The most interesting thing in Alien, which prefigures what would be the central subject of Blade Runner—Ridley Scott's next film that came just three years later—is that an artificial intelligence (Ash) could pass for a human. Ridley Scott no doubt already had replicantsFermerUltra sophisticated androids in the film Blade Runner (Ridley Scott, 1982), inspired by the novel Do Androids Dream of Electric Sheep (Philip K. Dick, 1966). In these works, replicants are distinguished from human beings by way of the Voight-Kampff test, which measures potential empathy. Ash (Alien) and David (Prometheus, Alien: Covenant) would no doubt have struggled to pass it, but it would probably be a formality for Call (Alien: Resurrection). in mind when he directed Alien. In the end, the saga's central figure, the most ambiguous and complex—and no doubt most terrifying—is perhaps not the xenomorph alien, but actually the android.
Further reading
L’Art et la science dans Alien, Frédéric Landragin, Roland Lehoucq, Christopher Robinson, Jean-Sébastien Steyer, éditions la ville brûle, published on 6 September 2019.
- 1. Linguist specializing in natural language processing and dialogue between humans and machines. He is a CNRS Senior Researcher at the laboratoire Langues, Textes, Traitements informatiques, Cognition (CNRS/ École normale supérieure /Université Paris-3 Sorbonne nouvelle).
Explore more
Author
Science journalist, author of chilren's literature, and collections director for over 15 years, Charline Zeitoun is currently Sections editor at CNRS Lejournal/News. Her subjects of choice revolve around societal issues, especially when they interesect with other scientific disciplines. She was an editor at Science & Vie Junior and Ciel & Espace, then...