You are here
Simulation and Modeling: Less is More
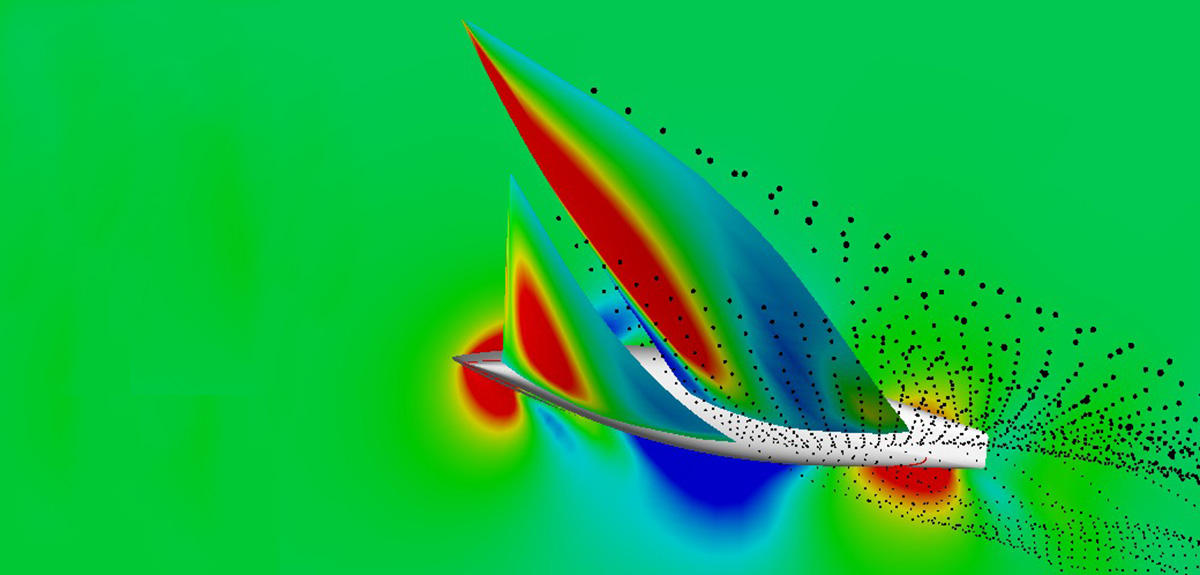
Frédéric Alexandre, as a researcher at the LaBRI1 you gave a lecture at the symposium “Modeling: Successes and Limitations” on December 6, 2016. What do the terms “modeling” and “simulation” mean in today’s research?
F.A.: Modeling and digital simulation refer to quite a specific problem, in that the use of digital technology has been widely developed in all fields whose phenomena can be represented by equations that can then be manipulated by computer. This is called “knowledge modeling.” This approach has become even more widespread in recent years due to the possibility of using big data and machine learning to generate statistics, in which case we talk about “representation models.”
Yet making a physical mockup and putting it in a wind tunnel is also a form of modeling and simulation. Building a model is a distinct process: it involves choosing a theoretical framework, a formalism for describing the object to be studied, and making sure that the whole set is adapted to the question concerning that object. It also means specifying, from the initial conception phase, the means of validating the model—proving that it corresponds to the issue being investigated. The simulation is the computerized implementation of the model, both in terms of software (in particular using computation schemes) and hardware, through an architecture adapted to the calculations to be carried out. This can be a combination of specific processors such as graphic processors, clusters of homogeneous machines, or a set of heterogeneous, possibly remote resources, called the “grid.” It should also be noted that managing this hardware requires the use of so-called “middleware” programs. At this stage, the simulation consists in varying the parameters to see how the model changes.
What is the connection between these concepts and those of theory, discovery and proof?
F.A.: Unlike data, which are simple observations of the object under study, a theory seeks to provide explanations concerning that object. When a theory cannot be proved by simple logical deduction, as is most often the case, it can be implemented using a model—and, possibly refuted experimentally through simulations. After refutation the model must be modified and, perhaps, a new theory proposed and corroborated through new tests. It is worth noting that the model and its underlying theory are continuously fine-tuned through a series of experimental adjustments—without ever reaching a “definitive truth.” As the epistemologist Karl Popper postulated, a scientific theory must offer an explanation—the best available at a given time—for observed phenomena but it must also provide the conditions for its own refutation.
How has this modeling/simulation approach changed the way research is conducted in certain disciplines?
F.A.: The availability of increased computing power and software-assisted simulation has made the modeling-simulation loop easily accessible. Perhaps too easily… For example, in the 2014 film Interstellar, it was thought to be more convenient to use physical simulations to represent giant waves. The risk in this case is to produce fast, easy simulations without questioning the validity of the associated models. Since the release of Interstellar this has led to endless debates among physicists about the choice of initial conditions used for the simulation.
There is a tendency to think that carrying out increasingly effective simulations requires ever more powerful computers, but is this actually the case? And can computing power be increased indefinitely?
F.A.: Not necessarily—progress in simulation is also due to improvements in the implementation software, allowing the programs to make much more efficient use of the hardware architectures. In the past 15 years, increased computing speed has been as much the result of the progress achieved in linear algebraic algorithms as of the growth in processor power.
For some time now we’ve been hearing about the end of Moore’s Law, which predicts regular increases in computing power. The argument could be based on the fact that we already seem to be reaching this stage, or that human ingenuity will always find alternative solutions. But I think it’s more important to know whether we should be developing increasingly complex models to the detriment of a reflection on the nature and pertinence of the models themselves. Besides, there’s the economic and ecological cost of operating computer clusters… Finally, and perhaps even more importantly, pushing a model beyond its validity range doesn’t make it more valid!
A simpler but better-adapted one is always preferable. While the increased use of simulations is justified to implement a model over a longer time period and across a larger space, or when it comes to testing more sets of parameters, caution is necessary when changing scale or aggregating several models, for example.
In order to represent reality more accurately, is it better to simplify models and simulations or make them more complex?
F.A.: This difficult question requires introducing another element. In addition to the theoretical models associated with digital simulations, we now have the combination of big data and machine learning, which makes it possible to analyze huge bodies of information through automatic learning procedures based on statistical models. In automatic language processing, for example, it is now more efficient to perform a statistical analysis of millions of sentences—rather than try to perfect language models—in order to develop effective automatic translation systems. Presumably, the same will soon be true for the description of physical objects, for which using the underlying physics equations will be less effective than analyzing a body of examples.
Without denying these systems' very real and even impressive performance , it is worth bearing in mind that they take the logic of computing power as far as it can go, to the detriment of analyzing the object under consideration, although an analysis may have led to a more elegant, and mostly more meaningful solution. Giant models with long lists of parameters can accommodate a lot of data without revealing their underlying logic. As René Thom puts it, predicting is not explaining.
Above all (to answer the question at last!), these two approaches, both statistical and theoretical, coupled with the massive use of simulation, sometimes lose sight of the bigger picture: what is the question and is this the best model for answering it? While these “mass” approaches can be well-suited and are now proving very effective for relatively regular phenomena, it is quite often more appropriate, for issues involving human, social, political or cognitive considerations, to formulate the questions carefully and define a simpler model, rather than immediately pressing the “red button” of simulation.
- 1. Laboratoire Bordelais de Recherche en Informatique (CNRS / Université de Bordeaux / Bordeaux INP).
Author
Lydia Ben Ytzhak is an independent scientific journalist. Among other assignments, she produces documentaries, scientific columns, and interviews for France Culture, a French radio station.