You are here
Modelling the lungs for personalised medicine
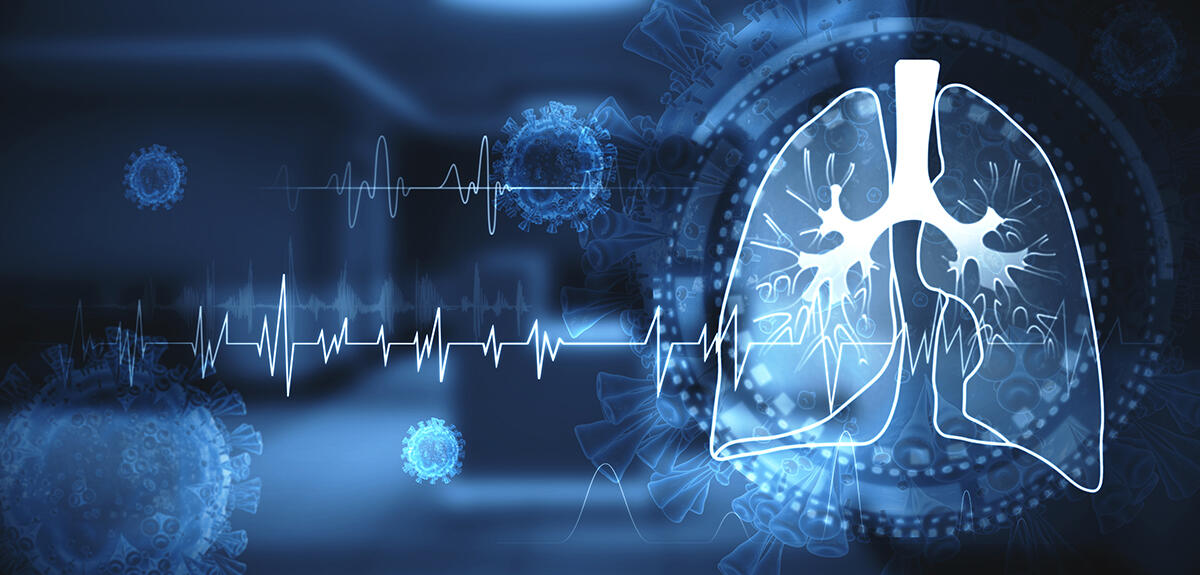
Today’s lung models are essentially single-scale and/or linear. However, the lungs are extremely complex organs, with strongly related phenomena occurring on different scales: for example, the lungs do not double in volume when twice the pressure is exerted on them. Martin Genet, an academic at École polytechnique, and his MEDISIM team at the Solid Mechanics Laboratory1 are developing new multi-scale, non-linear models and algorithms to generate more refined simulations of the lungs. The goal is to gain a better understanding of pulmonary fibrosis, as well as to promote medicine that is more personalised, objective, and quantitative. This research is funded by the French National Research Agency (ANR), whose goal is to support French research excellence and innovation at the national, European, and international level.
“Some idiopathic diseases, in other words whose causes and evolution are poorly understood, could have early warning signs that cannot be detected with current diagnostic approaches,” emphasizes Genet. “For instance, a model that can accurately estimate the rigidity of alveoli could improve our understanding and classification of different fibroses.” Thanks to their algorithm, the researchers can produce a faithful, precise, and personalised model, an actual “digital twin” of the organ. This algorithm is by necessity non-linear, which makes it more complex, but that is not the only difficulty researchers face in its development.
“Modelling is also a question of optimisation,” points out Genet. “Integrating physical phenomena is important, but it is not necessary to model all of the physics down to the lung’s atoms. You have to set limits to the approximation.” These optimisations allow for algorithms that consume less energy and computing resources, they can also have a direct impact on medical examinations. “If our algorithm remains effective with half the data, image acquisition time will be reduced by 50% as well, and hence the amount of radiation exposure during checkups.”
“Explainable AI” for optimised treatment
The MEDISIM team’s approach of coupling modelling and data shares similarities with the neural networks used by most of today’s AI, except that it requires much less information, but also more work to develop a specific model of the physics involved. Being able to operate with little input is a requirement of the medical sector: the model must include enough parameters to be precise and realistic, but not too many in order to be simulated quickly. Its development therefore entails a balance between these two necessities.
These multiple constraints nevertheless give this algorithm a rare advantage, namely that it is “explainable”. When a deep neural network is trained via machine learning on a large dataset, most of the time it is impossible to obtain precise details on how it functions, and hence to understand where its results come from. Digital twins “are based on a fine grasp of the physics involved, and each parameter has an explicit physical sense. This is a strength because we can understand the entire process that led up to the results”, stresses Genet.
The MEDISIM team’s algorithm can not only offer refined and precise models of a patient’s lungs – and create a “digital twin” – it can also provide its users with comprehensible decision-making, thereby helping to optimise medical examinations by reducing doses or examination times.
- 1. LMS (CNRS / École polytechnique).