You are here
Modeling Epidemics: Back to School
Despite huge progress in medicine and public health in the twentieth century, infectious diseases continue to kill millions of people every year. It is therefore vital to elucidate how such diseases spread so as to determine and evaluate appropriate ways of fighting them.
This virus is going viral
Concerning prediction, much has been said about social networks and how useful they can be when it comes to predicting the spread of epidemics, especially seasonal flu. More generally, it is essential to feed data describing human behavior on various scales into the models that characterize the spread of infectious diseases. The Gleam model, for instance, relies on detailed information on population densities and traveller flows between geographical regions to foresee the spread of a possible worldwide epidemic. In coordination with the WHO, it was used during the Ebola outbreak to carry out real-time assessment of the risk of introduction of the virus into different countries. Combined with data from Twitter, it is also used in fluoutlook.org to anticipate seasonal flu transmission.
These models describe disease dissemination at the medium and large scale, and therefore depend on precise data about human mobility on such scales. However, they are based on a relatively simplistic assumption about disease-causing contacts within a population, namely that all individuals can a priori interact with each other (a hypothesis called "homogeneous mixing").


This assumption is used for two reasons: on the one hand, the need for simple models whose results can be interpreted easily, as well as the difficulty of setting up models that incorporate several scales of description; and on the other, that of obtaining real data describing contacts within a population. However, the latter obstacle may soon be overcome.
From macro to micro data
A large proportion of the information regarding contacts between people comes from questionnaires, which are sometimes disseminated on a large scale and are relatively expensive. In the past few years, several research groups have developed infrastructures based on small wireless sensors that interact over short distances and can detect the physical proximity and face-to-face contacts of the individuals wearing them. One of the pioneers of this type of system, the French-Italian collaboration SocioPatterns1 has used it to collect data in widely varying contexts across several countries.
The doctor won’t see you now
In a series of scientific publications, the members of SocioPatterns describe the numerous results they have obtained from the collected data. Their analysis reveals that these networks are generally structured very differently from "homogeneous mixing." For instance, the pupils of a primary school spend around three times as long with children in the same class as with those in other classes; in hospitals, nurses have many contacts with each other and with patients, whereas doctor-patient and patient-patient interactions are scarce. The times of day when they take place also vary according to the context: they are scattered throughout the day in offices, but are predominantly restricted to breaks in schools, and depend on the organization of work and visits in hospitals.
Despite such differences, characteristics common to all these contexts can be observed, especially concerning the large differences in the length of contacts: most are short (under two minutes), whereas a significant number of interactions are much longer than average. Now, it is generally assumed that the likelihood of disease transmission is proportional to the length of contacts. Some are therefore far more important than others, and they cannot all be treated as being equivalent. Concerning their duration, more surprisingly, the similarity between statistics observed in various contexts and at different times shows that measurements carried out in one context can be used to produce a model that is reliable in another.
Partial recall
The combined use of sensors and questionnaires has also revealed significant discrepancies between the data collected by the two methods: a large proportion of short contacts are not reported in the questionnaires, unlike sufficiently long ones; in addition, the number of interactions tends to be underestimated, while their length is overestimated. Such differences can have a serious impact on models, and show the need for modellers to have a good knowledge of the source of the data used and of any possible bias.
Such data, although interesting in its own right, can above all be integrated in models of infectious disease spread. To do this, it is essential to understand which characteristics are most important to measure, and what level of detail is required. For example, precise information about the exact time when one individual is in contact with another is closely connected to a given context and time, and therefore of little use for a general model. On the other hand, a comparison of contacts outside and inside a class is relevant when it comes to determining how to contain an outbreak in a school.
Moreover, it has been shown that such information can even be extrapolated from incomplete data.2 Our work in schools has enabled us to propose and assess mitigation strategies in the event of an epidemic: although closing schools is considered to be an effective means of limiting disease spread, it is a costly measure. Since contacts mostly take place within classes, we have suggested reactively closing only those classes where cases of the disease have been detected. This is nearly as effective as closing the whole school, and considerably less expensive.3
Refining models, in real time
This type of analysis is still in its early stages. Data of this kind should continue to be collected in different contexts and made available to the scientific community. Many avenues remain to be explored, such as the development of methods that can find patterns in the information gathered (groups that have more contacts than others, sequences of contacts correlated in time): even if such methods appear highly theoretical, they could have significant applications in terms of public health.
It would also be important to enrich this data with other aspects of human behavior (such as hand hygiene) or microbiological samples that could help shed light on the factors that determine transmission events. To refine predictions and make them more reliable, multiscale models that combine different types of data (patterns of contacts between individuals within a building, mobility within and between cities) need to be designed, while only retaining relevant characteristics for each scale and type of data.
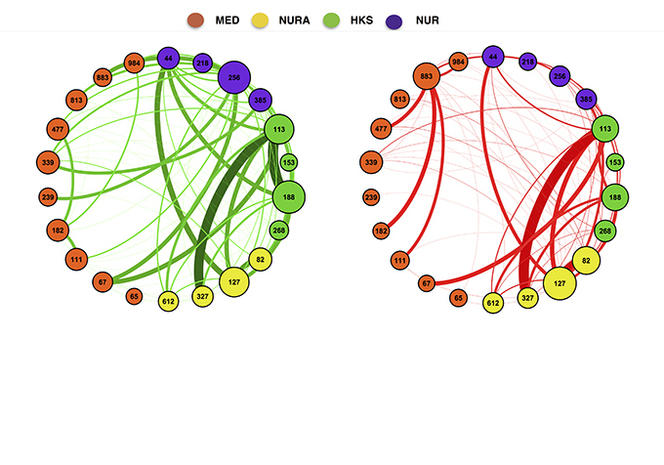
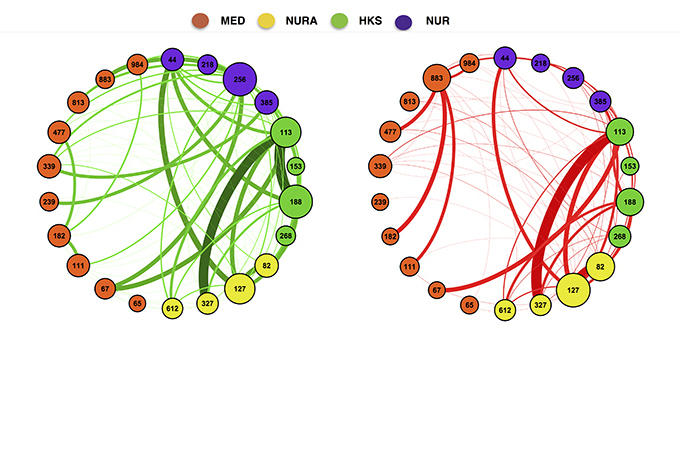
We could even go further by incorporating the reactions of individuals to the fact that an epidemic is underway, which might lead to a spontaneous reduction in contacts or mobility. This field is still very much open, and requires the integration of epidemiology, modeling, and social science skills.
The analysis, views and opinions expressed in this section are those of the authors and do not necessarily reflect the position or policies of the CNRS.
- 1. SocioPatterns is a collaboration between researchers and developers from the ISI Foundation (Italy), the CNRS-Centre de Physique Théorique (France), ENS Lyon-Laboratoire de Physique (France), and the company Bitmanufaktur (Germany).
- 2. M. Génois et al., "Compensating for population sampling in simulations of epidemic spread on temporal contact networks," Nat. Comm., 2015. 6: 8860.
- 3. V. Gemmetto et al., "Mitigation of infectious disease at school: targeted class closure vs school closure," BMC Infectious Diseases, 2014. 14: 695.