You are here
Human vs Machine: It's Go Time
In a match last October, the AlphaGo program developed by Google's "DeepMind" subsidiary beat, 5 games to 0, the French professional player Fan Hui,1 who is ranked 2 dan (on the professional scale from 1 dan to 9 dan) and is today Europe's best player. The story was related by the journal Nature.2 This was the first time that a computer beats a professional player. But in the world of artificial intelligence, the progress demonstrated by the AlphaGo victory wasn't expected for another ten years or so.
The moment of truth, however, will take place between March 9-15 in Seoul, where AlphaGo will face the South Korean Lee Se-dol, who is 9 dan, and is considered the best player in the world as well as a Go living legend. This new game, which will be broadcast live on the Web, comes with a $1,000,000 prize for the human champion if he wins.
A game of Chinese origin involving two players, Go is played on a board, called the goban, using black and white pieces, called stones. The players place their stones in turn on the intersections of the goban, which is made up of 19 by 19 lines. The objective is to occupy the largest territory possible. Despite very simple rules, this game is much more difficult to master for a computer than checkers or chess (whose champion G. Kasparov was beaten by Deep Blue in 1997). It is partly the colossal number of configurations possible in Go games— there are 10170 legal positions, that is 1 followed by 170 zeros, as opposed to 10120 in chess— which makes the game so complex for artificial intelligence.
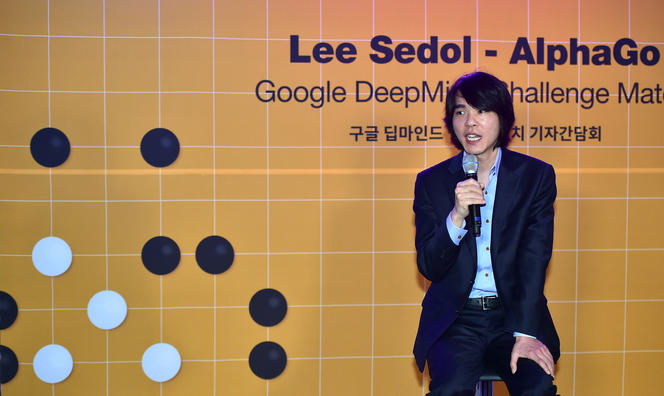
A few months ago, the Japanese program Zen was considered the world's best software, but was far below the level of the best professional players. Its very classical principle is based on evaluating the suitability of moves by simulating thousands of games.
How does it work?
This approach, which dates back to 1993, was notably improved in 2006 thanks to the Monte-Carlo "tree search" method, in which games are simulated based on the current configuration in a game. However, the program does not simulate all possible games, only specific ones. In fact, a large number of game endings are played "at random" based on the current position (this is the "exploratory" phase of the tree of possibilities), and then the proportion of winning versus losing games is counted. This statistical estimate is gradually refined by prioritizing the number of good moves.
AlphaGo also uses this exploratory technique, which enabled French researchers to score the first wins against professional players in 2007-2013 (the Tao team at Inria Saclay, and then Rémi Coulom in Lille—these games were either on the small 9x9 Goban or with handicap contrarily to AlphaGo which played even games). The DeepMind program, however, adds other tools, beginning with neural networks, the "in silico" equivalent of biological neurons. The neurons "learn" to connect themselves in the best way based on the results yielded during pre-game simulations, while the exploratory phase of the Monte-Carlo method is conducted during the game (one can for instance choose to give the program 20 seconds to conduct the exploration before determining its move).
A neural network learns through imitation, by reproducing Go matches between expert human players. Researchers in Edinburgh have shown that the only limit to these methods was computing power—the greater this power, the better the neural networks played. And AlphaGo has accumulated 30 million moves played by professionals!
AlphaGo's neural network also makes progress by playing against itself. The method has been known since 1992, and makes use of reinforcement learning. This is where Google's phenomenal computing power is useful.
Finally, a second neural network studies the program's earlier matches against itself, and thus learns to recognize "good" situations to guide the random exploration. This is called supervised learning, in which the computer uses examples to try to distinguish good situations from bad ones. There are thus two neural networks that guide the random exploration.
What purpose does this serve, other than playing Go?
The use of neural networks is not new, but DeepMind took the technique much further, with immense computing power and numerous layers of neurons (the deep learning), and cleverly integrated it into the random exploration technique. It is this combination of different techniques that is innovative, at least with respect to Go. The DeepMind program even took the luxury of playing what is called an "empty triangle" during one match. This move, usually considered a very bad one, can in rare cases prove to be apt, and AlphaGo was able to astutely risk it.
Imitation learning is widely used in robotics, because it makes it possible to reproduce gestures provided by human experts. It is not simply a question of copying human gestures, but learning to generalize them. The problems of control (control of vehicles, military applications such as the planning and execution of missions by the infantry3) also often call on reinforcement learning.
Finally, supervised learning is the most effective tool for recognizing images. If you have a program that can detect (well) if there is a pig in your photo or video, it is probably using supervised learning on neural networks. The use of what are called convolutional neural networks, which are very similar to those found in the visual cortex, has in particular been known since 1998 with the work of Yann LeCun. Convolutional networks, by applying the same neurons for all parts of the image, make it possible to recognize a pig whatever its position in the image.
Predictions?
The 5 to 0 victory against Fan Hui was impressive, but he is ranked in the top 600 to 700 players in the world, and thus far from world-class level. Moreover, experts indicate that the human initially led for a large part of the match before being defeated, and that a stronger player, such as Lee Se-dol, would have won comfortably. The computer engineers at DeepMind themselves recognize that the program that played against Fan Hui would not have won against Lee Se-dol, but they maintain that their current program is much more powerful. Only they have the means to test it before the crucial encounter. Their (optimistic) prediction could thus prove reliable.
The analysis, views and opinions expressed in this section are those of the authors and do not necessarily reflect the position or policies of the CNRS.
- 1. Fan Hui, who is of Chinese ancestry, was naturalized as a French citizen in 2013.
- 2. http://www.nature.com/nature/journal/v529/n7587/full/nature16961.html(link is external)
- 3. http://www.lamsade.dauphine.fr/~cazenave/papers/icaps2013.pdf(link is external)